In recent years, the high costs, time-consuming processes, and high risks of failure in clinical trials have underscored the urgent need for innovation. As pharmaceutical companies strive to bring effective drugs to market faster and more efficiently, AI and machine learning have emerged as transformative tools. This article explores how these technologies are reshaping every phase of clinical trials, from patient recruitment to data management and personalized treatment design. With real-world examples and case studies, we delve into how AI and ML are improving efficiency, safety, and outcomes in clinical trials, setting the stage for a future of more adaptive, patient-centric trials.
In the pharmaceutical drug development cycle, nearly half of the average time and cost is dedicated to clinical trials. These research studies, conducted on human participants, evaluate the safety and efficacy of medical interventions. In this process, a large patient group tests new treatments to confirm effectiveness, monitor side effects, and compare new drugs with existing options.
Failed trials can result in losses of $800 million to $1.4 billion per study. Traditionally, this occurs for two key reasons: the difficulty in selecting and recruiting the right patients in time and the lack of proper technology to manage operations. As a result, the number of new drugs reaching the market has been steadily declining, even though pharmaceutical companies are investing more in R&D.
In modern clinical trials, the integration of AI and ML is enhancing the efficiency and precision of trial design, enabling faster patient recruitment, real-time data analysis, and more personalized treatment approaches. In this article, we break down the role of AI and ML in clinical trials with special attention to use cases.
Machine Learning and AI in Context of Clinical Trials
AI helps overcome the challenges of clinical trial design, streamlining complex tasks such as patient selection, trial monitoring, and data analysis. ML models predict potential risks and adverse events early in the process, allowing for quicker interventions and enhancing patient safety throughout the trial. Moreover, intelligent systems optimize trial protocols by simulating various scenarios, predicting outcomes, and adjusting trial parameters in real time.
ML and deep learning (DL) in particular are able to automatically find patterns of meaning in large datasets such as text, speech, or images. Natural language processing (NLP) can understand and correlate content in written or spoken language, and human-machine interfaces (HMIs) allow natural exchange of information between computers and humans.
These capabilities can correlate large and diverse datasets to improve patient-trial matching and recruitment before the trial begins. During the trial, they enable automatic and continuous patient monitoring, improving adherence control. This leads to more reliable and efficient endpoint assessments.
The Use of AI and ML in Clinical Trials
AI is significantly transforming clinical trials. For example, Twist Bioscience uses AI models like AlphaFold2 for protein structure prediction; as a result, they improved prediction speeds by up to 82%. The Nobel Prize 2024 in Chemistry was awarded to three scientists for their work with AlphaFold, a neural network AI program designed to predict the complex folding patterns of proteins and even create new proteins. The recipients—Demis Hassabis and John Jumper from Google DeepMind, along with David Baker from the University of Washington—have highlighted the program’s remarkable potential for advancing drug discovery and development. This award emphasizes the importance of the use of AI in modern medicine. Let’s dive into the most complex examples of AI-driven tools usage in clinical trials.
Patient Recruitment and Selection
Predictive models leverage AI and ML to analyze vast datasets, identifying patterns that can help determine which patients are most likely to benefit from or meet the criteria for specific trials. The integration of EHRs takes this a step further by allowing real-time access to comprehensive patient data, such as medical history, diagnoses, and treatments. By matching this information with trial requirements, EHR-based systems can quickly and accurately identify eligible participants.
TrialGPT helps assess each criterion individually and consolidates the results to provide an overall prediction for trial suitability. In tests across three publicly available cohorts of 184 patients, with over 18,000 trial annotations, TrialGPT’s performance was benchmarked against human experts. Additionally, three physicians labeled over 1,000 patient-criterion pairs to measure criterion-level accuracy, where TrialGPT achieved 87.3% accuracy, nearly matching expert levels (88.7%-90.0%). TrialGPT’s overall scores were highly aligned with human judgments and outperformed competing models by 32.6% to 57.2% in trial ranking and exclusion. In real-life clinical trial matching, it reduced screening time by 42.6%. These findings highlight the potential of large language models (LLMs) like TrialGPT for improving trial matching.
Data Management and Integration
The volume and complexity of data encompass everything from EHRs and genomic data to wearables and real-time monitoring systems. Ensuring data quality and consistency is another key challenge in clinical trials. Through automated processes, AI helps detect anomalies, fill in missing data, and maintain consistency across integrated data sources. This reduces the likelihood of errors and ensures that all trial participants’ data is uniformly interpreted.
Twist Bioscience partnered with Oracle Cloud Infrastructure (OCI) to enhance its data management and integration capabilities, focusing on protein structure prediction using advanced AI models like AlphaFold2, ParaFold, and ESMFold. Oracle’s high-performance GPU instances and cloud storage solutions enabled Twist to significantly improve the efficiency and speed of its AI models, achieving up to an 82% increase in prediction speed and notable cost savings. This collaboration also set the stage for future innovations in DNA-based digital data storage, demonstrating Twist’s potential to revolutionize data archiving on an unprecedented scale.
Monitoring and Adverse Event Detection
Adverse drug events (ADEs) are a leading cause of healthcare-related harm. In clinical trials, the integration of AI for monitoring and detecting ADEs presents significant potential for improving patient safety. For prediction, AI models provide dosing recommendations or suggest additional interventions to prevent ADEs in trial participants.
Each year, global life science company Bayer processes hundreds of thousands of AE reports from various sources, including phone calls, emails, its customer relationship management system, and other channels. Much of this data is unstructured, often in free-text format, and frequently arrives through platforms not specifically designed for AE reporting. One team, for example, reviewed 10,000 records daily, with up to 800 needed to streamline this process. The AE-reporting engine has two endpoints: one for high-throughput batch inference and another for low-latency client interactions.
Using AWS, Bayer’s pharmaceuticals division developed a centralized AE-detection engine that leverages ML to streamline the identification and reporting of adverse events. This engine enables near real-time inference via APIs, allowing multiple systems to access it without custom integration, with an average response time of 170 milliseconds for immediate feedback scenarios like AI chatbots. The batch inference option processes multiple files simultaneously, significantly reducing processing time and allowing users to concentrate on relevant information.
Precision Medicine and Personalized Treatment
Precision medicine focuses on tailoring treatments to the unique characteristics of each patient, including genetic makeup, lifestyle, environmental factors, and clinical histories. ML algorithms can evaluate genetic data alongside clinical histories to forecast how a patient will respond to specific therapies. For example, supervised learning methods like regression analysis or support vector machines can be trained using past patient data to predict how likely a therapy is to succeed. DL models, particularly convolutional neural networks (CNNs), can process genomic data and imaging studies to detect subtle biological markers for a patient’s likelihood of responding to a particular drug.
In a study evaluating the use of Watson for Oncology (WFO) for lung cancer patients in China, researchers found that WFO’s treatment recommendations were consistent with those of a multidisciplinary team in 65.8% of cases. The study included 182 patients, with 149 receiving WFO-supported recommendations; however, 18.1% of cases were unsupported by the AI system. Factors such as cancer stage and pathological type significantly influenced the consistency of WFO’s recommendations. The findings suggest that WFO is useful as a supplementary tool but cannot replace oncologists, emphasizing the need for further adaptation to local medical practices and patient characteristics.
AI Medical Image Analysis
Advances in artificial neural networks, particularly CNNs, have significantly enhanced image processing by automating feature extraction, leading to efficient, end-to-end lab management systems. Vision Transformers (ViTs) further improved on CNNs by introducing the ability to handle long-range dependencies and positional information in images through self-attention mechanisms, offering enhanced performance in tasks like classification and segmentation. Generative models, such as GANs and VAEs, have revolutionized data augmentation in medical imaging by generating realistic synthetic data, improving model training where data is scarce. Lastly, deep learning performance in multimodal image classification can be optimized using specialized techniques for data preprocessing, feature alignment, and model regularization.
Automated Lab Tests
One of the key components of automated lab tests is the implementation of high-throughput screening (HTS). It enables the simultaneous analysis of thousands of samples, utilizing robotic systems to automate the preparation, execution, and data acquisition phases of laboratory testing. Imaging systems can quickly analyze sample reactions, providing real-time feedback on biological interactions. For instance, predictive modeling can determine how specific biomarkers correlate with treatment efficacy, allowing for more targeted patient recruitment and monitoring.
Research demonstrates AI’s strong potential in a laboratory automation domain, particularly through its ability to enhance diagnostic accuracy and efficiency. For example, a DL model achieved 87% sensitivity and 92% specificity in distinguishing COVID-19 from other lung diseases, with an impressive area under the curve (AUC) of 0.95. AI algorithms have also shown remarkable precision in identifying infectious diseases, such as the 95% early detection rate of skin cancer using the Skinvision app. Additionally, AI-driven laboratory systems have achieved diagnostic accuracy with mean AUCs of 0.98 and 0.94 in lab tests, significantly improving the speed and reliability of diagnoses. These advancements underscore AI’s potential to revolutionize laboratory operations by optimizing resource use.
Trial Design and Optimization
By leveraging ML algorithms and predictive analytics, researchers can create more robust study designs that account for variability in patient responses and optimize trial parameters. For instance, simulation models such as discrete event simulation (DES) allow trial planners to explore various scenarios by mimicking the progression of patients through the trial. By inputting parameters like enrollment rates, dropout rates, and treatment effects, researchers can visualize potential outcomes. This iterative process helps in refining inclusion and exclusion criteria to ensure the recruitment of an appropriate patient population.
Moreover, AI-driven optimization techniques can automatically adjust key trial parameters, such as sample size, dose regimens, and treatment duration, based on real-time data. For example, reinforcement learning algorithms can analyze interim results and adapt the trial protocol to maximize efficacy while minimizing risks.
The development of a Clinical Trial Simulation (CTS) tool for Alzheimer’s disease (AD) demonstrated significant advancements in optimizing clinical trial design using AI. This tool integrates diverse data sources, including patient-level data and literature, to model disease progression, drug effects, and trial features, facilitating more accurate and efficient trial designs. By simulating various scenarios, such as symptomatic and disease-modifying drug effects, the CTS tool can optimize trial parameters like sample size and duration, improving power and reducing bias. Regulatory agencies, including the FDA and EMA, endorsed the tool, recognizing its scientific validity and utility in future clinical trial designs.
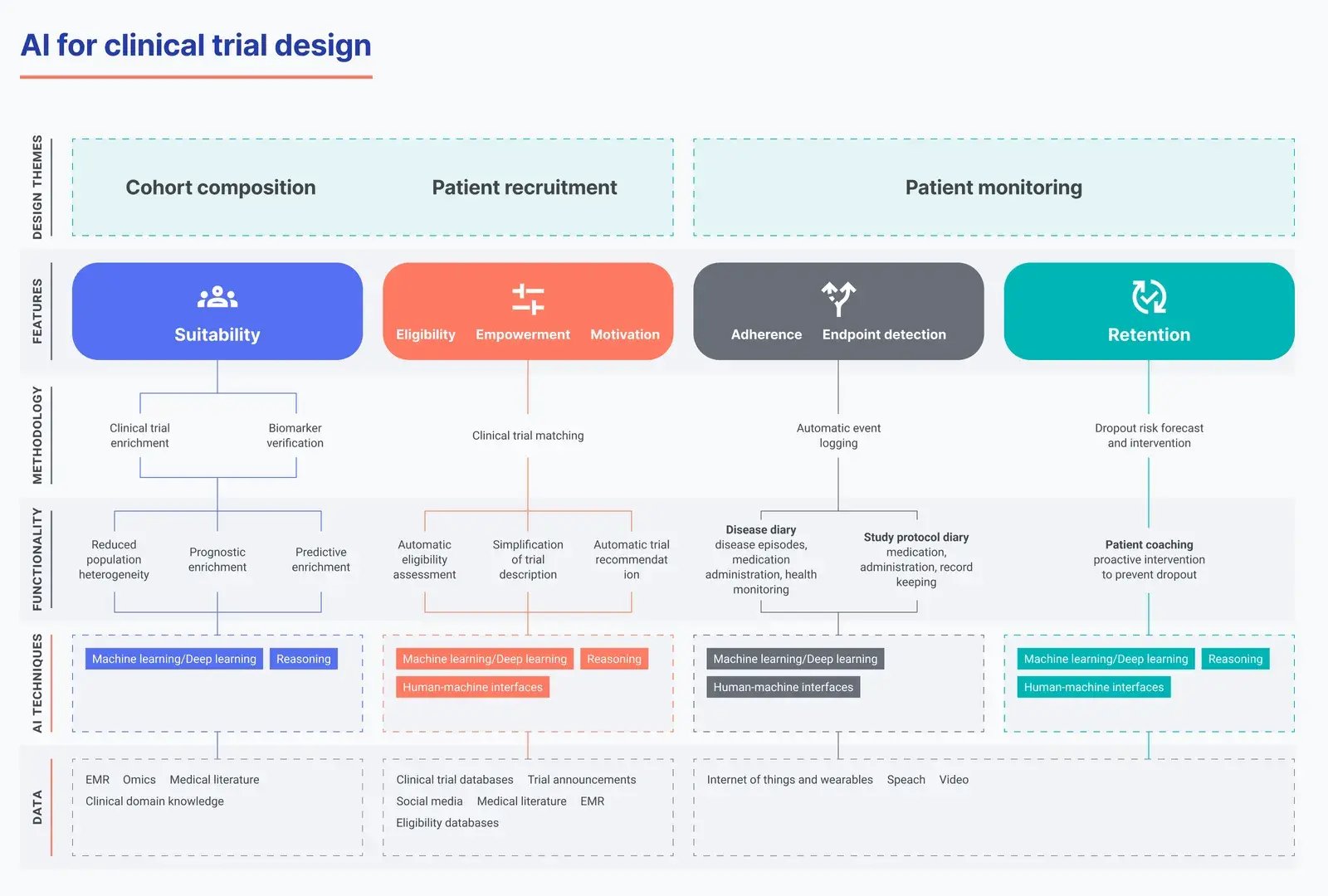
Benefits of AI and ML in Clinical Trials
The integration of AI into clinical trials offers numerous advantages that are transforming the overall conduct of clinical research. ML models further enhance efficiency by continuously refining their algorithms as new data becomes available. Below are the key benefits AI and ML bring to clinical trials:
Increased Efficiency
AI accelerates the recruitment process, quickly identifying suitable candidates for trials and significantly reducing the time and costs associated with various trial phases. Automating tasks such as patient matching and data entry reduces human labor and expedites overall trial timelines. Algorithms can analyze patient databases to identify suitable candidates quickly, streamlining the selection process and ensuring trials begin without delay.
Data-Driven Insights
The incorporation of AI in clinical trials leads to enhanced data analysis capabilities and improved prediction accuracy. ML models can sift through vast datasets to identify patterns and correlations that may be overlooked by human analysts, effectively reducing human error in data interpretation. This results in more reliable insights, allowing researchers to make informed decisions throughout the trial process.
Accelerated Data Analysis
With AI, vast amounts of data can be processed and analyzed far more quickly. This allows researchers to identify trends, correlations, and actionable insights at a much faster rate, speeding up the decision-making process and enabling real-time adjustments to trial protocols. ML algorithms accelerate this process by learning from the data, allowing for the discovery of trends and correlations in real-time, improving the adaptability of clinical trials.
Better Patient Outcomes
Personalized treatment plans powered by AI result in more effective therapies and improve patient outcomes. AI also aids in the early detection of potential adverse effects, ensuring timely interventions and improving overall patient safety. ML can also predict how patients will respond to specific treatments, allowing for more tailored and effective care strategies.
Enhanced Decision-Making
Data-driven insights and predictive analytics from AI lead to more informed clinical decisions. These tools optimize future trials and drug development processes, ensuring that decisions are supported by comprehensive data analysis. ML enables the prediction of long-term trial outcomes, assisting in the early identification of successful treatments and potential pitfalls.
Optimize Clinical Trials with Coherent Solutions
Accelerate recruitment and analysis with our AI-driven expertise to improve patient outcomes.
Challenges and Considerations of AI in Clinical Trials
While AI offers significant benefits in clinical trials, it also presents several challenges that organizations must navigate to ensure successful implementation. In 2025, the U.S. Food and Drug Administration launched a groundbreaking initiative to phase out traditional animal testing in drug development. This policy shift encourages the adoption of AI-powered models and other New Approach Methodologies, such as organ-on-chip and in silico simulations, to assess drug safety and efficacy. The innovations are designed to accelerate clinical research timelines and improve ethical standards - marking a major step toward more humane and efficient drug discovery. Incorporating AI into clinical research processes is not merely a plug-and-play solution; it requires a comprehensive strategy that addresses various facets, from technology integration to data management.
The implications of AI adoption extend beyond technical considerations, impacting regulatory compliance, ethical standards, and patient trust. Organizations must be prepared to invest in training and resources to overcome these obstacles and promote a culture of innovation while ensuring patient safety and data integrity. Understanding these challenges is essential for organizations aiming to leverage AI effectively.
Cost and Implementation: The initial investment in AI technologies can be substantial, posing a barrier for smaller organizations or those with limited resources. Implementing these advanced solutions requires not only financial commitment but also time and expertise.
Data Quality: The effectiveness of AI-driven insights hinges on data quality. Poor-quality data can lead to misleading results, compromise patient safety, and reduce the overall effectiveness of AI models. Organizations must prioritize robust datasets for effective model training and validation.
Data Privacy and Security: Handling sensitive patient information necessitates strict adherence to data privacy regulations, such as GDPR and HIPAA. Ensuring compliance is critical to maintaining patient trust and avoiding legal repercussions.
Algorithm Transparency and Bias: Ensuring transparency in AI models is essential for gaining stakeholder confidence. Organizations must address potential biases in machine learning algorithms that could skew results or create inequities in treatment outcomes.
Integration with Existing Systems: Integrating AI solutions with current clinical trial infrastructure can present technical and operational hurdles. Organizations must ensure compatibility to maximize the effectiveness of their AI initiatives and streamline workflows.
Future Developments for AI in Clinical Trials
The future of AI in clinical trials is poised for transformative advancements, driven by the integration of cutting-edge technologies such as genomics and wearables. By combining AI with genomic data, researchers can develop more targeted therapies, enhancing the precision of treatments tailored to individual patient profiles.
Patient-centric trials will become increasingly prevalent, emphasizing real-time data collection, remote monitoring, and adaptive protocols. These innovations not only increase patient engagement and convenience but also allow for more responsive trial designs that adapt to participant needs and treatment responses.
Moreover, the development of explainable AI models will play a crucial role in fostering trust and transparency. By providing clear and understandable explanations for their predictions and recommendations, these models will empower healthcare professionals and patients alike, ensuring informed decision-making and enhancing the overall acceptance of AI-driven solutions in clinical research. As these developments unfold, AI will continue to reshape the landscape of clinical trials, paving the way for more effective and efficient healthcare solutions.
Coherent Solutions: The Reliable AI Partner for Life Sciences
Coherent Solutions delivers custom AI software to optimize clinical trials. With a team of 100+ AI and data analytics experts, we have a proven track record of success with Fortune 500 clients. Our advanced solutions enhance trial design, patient recruitment, and data management. Since 1995, Coherent Solutions has been a trusted leader in delivering expertise for life science projects. Partner with us for innovative AI-driven optimization.
FAQ: AI for Clinical Trials Design
-
AI and ML help optimize trial protocols, identify suitable patient cohorts, simulate outcomes using digital twins, and enhance decision-making throughout the trial lifecycle.
-
Benefits include faster patient recruitment, real-time safety monitoring, predictive analytics for better outcomes, cost reduction, and improved trial success rates.
-
In 2025, the FDA began phasing out mandatory animal testing, promoting AI-based models and New Approach Methodologies (NAMs) to streamline and modernize drug development.
-
Key concerns include algorithmic bias, data privacy, and the need for transparency in AI decision-making to ensure fair and ethical outcomes across diverse populations.