Data analytics and AI are now essential for businesses looking to stay competitive. This article will help you build a strong data analytics strategy. Whether starting fresh or refining your approach, you'll learn practical steps, how to create a roadmap, and best practices to make the most of your data and drive measurable business results.
Contents
Data Analytics and Its Importance
Benefits of Data Analytics Strategy for Business
Summary of the Roadmap Process
Key Questions for Strategy Development
Three Value Propositions for D&A
Best Practices for Implementing a Data Analytics Strategy
From Data Strategy to Action: Bridging Planning and Operations
Common Pitfalls and How to Avoid Them
According to a 2023 study, 91.9% of organizations reported measurable value from their data and analytics investments. A Deloitte survey of 29 Chief Data Officers (CDOs) revealed that 68% prioritized improving their use of data analytics, 61% focused on delivering on their data strategy, and 50% sought to enhance data culture in their organizations. The rapid adoption of generative AI further emphasizes the need for clear, value-driven data strategies. Gartner projects that by 2026, fostering data literacy and promoting cultural change will be essential for the success of Chief Data and Analytics Officers (CDAOs).
Organizations looking to establish and refine their data analytics strategy should foster a data-driven culture and use analytics to drive innovation. The guidance we provide below will help you unlock the full potential of your data.
Data Analytics and Its Importance
Data analytics refers to the process of collecting, analyzing, and interpreting data to extract actionable insights. It enables businesses to make data-driven decisions, optimize processes, and enhance customer experiences. A strong data analytics strategy is crucial for staying competitive and driving actions that foster business growth.
More than a tool, data analytics has become a vital asset. Organizations can identify emerging trends, address inefficiencies, and predict customer needs with well-structured data practices. Beyond immediate actions, data analytics supports strategic planning, equipping companies to adapt to market changes and drive innovation.
A well-defined big data analytics strategy amplifies this impact by leveraging vast datasets for deeper insights, driving both immediate improvements and long-term, transformative change. This approach enables companies to harness the full potential of large-scale data, aligning analytics with broader business goals to fuel sustainable growth and industry leadership.
Benefits of Data Analytics Strategy for Business
A comprehensive data analytics strategy not only optimizes operations but also cultivates a culture of innovation and resilience. Below are the key benefits that make data analytics an essential driver of business growth
Improved Decision-Making
Data-driven insights allow leaders to make more accurate decisions faster. This method empowers organizations to adapt quickly to changing circumstances.
Operational Efficiency
Analytics can identify inefficiencies and suggest improvements, leading to streamlined operations. Optimizing resources and processes helps reduce costs and boost productivity.
Competitive Advantage
By understanding market trends and customer behavior, companies can stay ahead of competitors. Using this information enables businesses to innovate and align their offerings with market needs.
Risk Mitigation
Predictive analytics help businesses anticipate and mitigate risks. By identifying potential threats early, organizations can implement proactive measures to minimize their impact.
Innovation
AI and ML foster innovation by providing insights enabling new products and services development. These technologies also help businesses optimize existing offerings and explore untapped opportunities.
Data Analytics Roadmap
Building an effective data analytics and AI strategy takes a step-by-step approach. Here are the five steps to help you implement and improve your strategy — from laying a strong foundation to adapting as new technologies emerge.
1. Create a D&A and AI Vision Strategy
The first step in any successful data and AI journey is to establish a clear vision and strategy that aligns with business goals and secures stakeholder buy-in.
Actions:
- Understand business priorities and how data can support them
- Position data as a key asset for digital transformation
- Optimize the D&A and AI investment portfolio based on risk, opportunity, and return
Deliverables:
- Vision documents
- Stakeholder presentations
- Strategy roadmap
2. Establish Operating Framework
The next step is to create an operating model that supports data literacy, AI readiness, and business value.
Actions:
- Cultivate data literacy across the organization
- Build advanced analytics capabilities
- Leverage AI and ML to drive business value
Deliverables:
- Balanced frameworks supporting agility
- Data governance
- Regulatory compliance
3. Cultivate Culture and Establish Governance
This step focuses on fostering a mindset that values data and AI, while also establishing governance structures that ensure data is used responsibly and aligns with business objectives.
Actions:
- Assess and improve data and AI literacy
- Establish a governance framework driven by business outcomes
- Incorporate ethical and legislative trends into governance practices
Deliverables:
- Governance structure
- AI ethics policies
- Compliance frameworks
4. D&A Value Management
In this step, the goal is to demonstrate the value of data and AI investments by linking them directly to business outcomes.
Actions:
- Integrate D&A with digital platforms for enterprise-wide agility
- Create a prioritized investment portfolio and track the value delivered
- Communicate the value of D&A to stakeholders, including the C-suite
Deliverables:
- Metrics
- Dashboards
- Value-tracking reports
5. Refine and Progress
The final step focuses on continuous improvement, ensuring that the strategy adapts to shifts in the business environment and maximizes its impact as new tools and capabilities emerge.
Actions:
- Track program effectiveness and seek feedback for refinement
- Reassess strategies based on emerging trends like IoT, generative AI, and data fabric
- Develop new skills and roles as the strategy evolves
Deliverables:
- Updated roadmaps
- Maturity assessments
- Revised processes
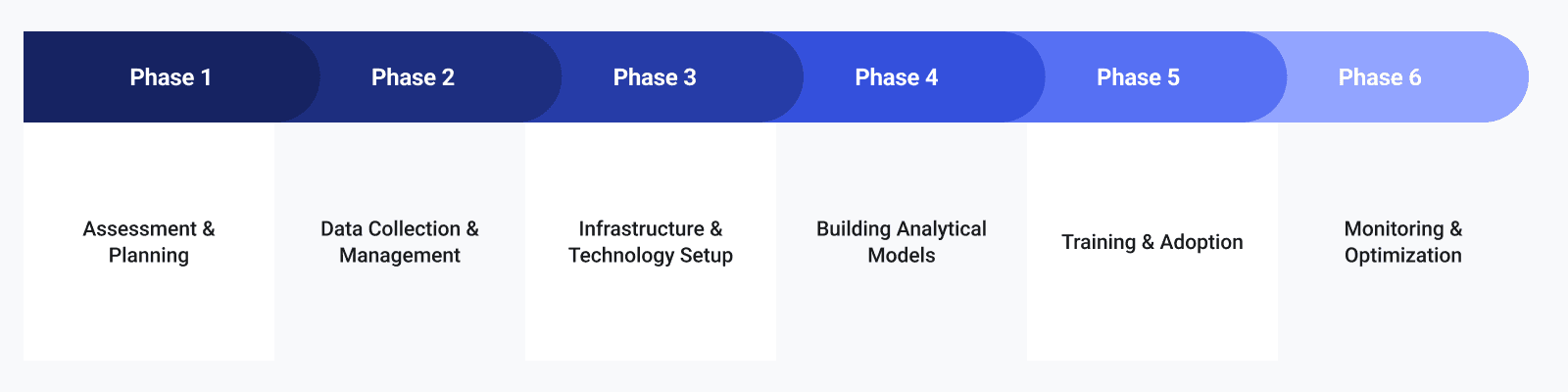
Overview of the Roadmap Process
Each stage of this roadmap is crucial for integrating analytics and AI into the core of business strategy, helping to drive significant outcomes. Designed as an iterative framework, the roadmap encourages continuous learning and adaptation in response to technological advancements. The foundation is a clear vision and strategy, aligning all efforts with business priorities. This is followed by an operating model that establishes the necessary skills and infrastructure for achieving strategic goals.
A phased, structured approach begins with defining data as a key transformative asset, progressing to develop organizational literacy and readiness for advanced analytics. Lasting value is built through a data-oriented culture, underpinned by governance that ensures ethical and responsible usage. Monitoring impact is essential, linking investments in analytics and AI directly to business performance through metrics and dashboards. Finally, the strategy’s ongoing refinement allows it to remain aligned with emerging technologies and evolving business needs, ensuring its continued effectiveness.
Build a Data Strategy for Real Results
Let us guide your data analytics adoption roadmap, turning raw data into actionable insights.
Key Questions for Strategy Development
A strong data, analytics, and AI strategy starts with key questions about scope, leadership, and goals. This will help you align efforts, engage stakeholders, and define success metrics.
1. Is the scope of the initiative strategic, operational, or governance?
A strategic scope aims at creating long-term value and transforming the business, while an operational scope enhances day-to-day processes for greater efficiency. Governance, on the other hand, centers on ensuring data control, compliance, and ethical use.
2. What are the key stages and activities of a data, analytics, and AI initiative?
Implementing a successful data, analytics, and AI initiative requires a structured approach. Each stage, from discovery to evaluation, is vital for aligning efforts with business goals:
- Discovery: Define strategic direction and identify business challenges
- Planning: Develop a roadmap and allocate resources
- Execution: Implement the strategy, train staff, and monitor progress
- Governance: Ensure data compliance and ethical use
- Evaluation: Measure results and refine the strategy
3. Which leaders and teams need to be involved?
Successful implementation of a data, analytics, and AI strategy requires collaboration across various leadership and technical teams. All contributors play a crucial part in the smooth execution of the strategy:
- CDAO: Leads strategy and alignment with business goals
- Chief Information Officer (CIO): Collaborates on technology infrastructure
- Data Governance Leaders: Establish policies for data security and compliance
- Software Engineers: Build data systems and pipelines
4. Who are your stakeholders?
Identify internal stakeholders, like teams and executives, who rely on data to enhance decisions and optimize operations. Include external stakeholders, such as customers, partners, and regulators, who are affected by or contribute to data initiatives. Mapping out each stakeholder’s role and interest ensures that their unique needs are incorporated into the strategy, helping to foster engagement and support for data initiatives across the organization.
5. What are the required business outcomes for each stakeholder?
For each group, define targeted outcomes that align with their goals and add value. For instance, executives may prioritize revenue growth and operational efficiency, while operational teams may focus on process optimization and customer service enhancements. This alignment allows each stakeholder to see the direct impact of data initiatives on their objectives, from cost savings and risk management to improved customer value and regulatory compliance.
6. How will the D&A strategy help achieve these goals?
Identify specific use cases, initiatives, and data products that directly support each stakeholder’s objectives. For example, predictive analytics might help sales teams better anticipate customer needs, while machine learning could optimize inventory management. By detailing how data and analytics will enhance each stakeholder’s workflow, the strategy demonstrates its practical, targeted benefits.
7. What key performance indicators (KPIs) will measure success?
Establish KPIs that reflect both progress and outcomes. Progress metrics, such as the percentage of employees trained in data literacy or the number of automated reports generated, indicate the adoption level and operational changes within the organization. Outcome metrics, such as revenue growth, cost reduction, or customer satisfaction scores, reveal the strategy’s tangible impact on business goals, providing stakeholders with clear evidence of success.
Three Value Propositions for D&A
Organizations can leverage data and analytics in different ways, each delivering unique value depending on strategic priorities. Whether serving as a utility to make data broadly accessible, as an enabler to support specific business outcomes, or as a driver of new revenue streams and innovations, each approach plays a distinct role in enhancing the business.
D&A as a Utility
This approach focuses on creating a broad-based capability that serves all stakeholders across various needs. Its primary output is a continuously available platform, accessible at any time by anyone in the organization.
Key success measures:
- Platform availability
- Ease and speed of accessing data
- Time taken to integrate new data sources or APIs
D&A as an Enabler
This method is designed to support specific business objectives by delivering customized, purpose-built solutions. The primary outcome is targeted improvements that directly enhance business processes.
Key success measures:
- Business KPIs such as improved conversion rates, cost savings from predictive maintenance, or enhanced fraud detection
- Clear link between D&A initiatives and business outcomes
D&A as a Driver
The focus is on driving new business goals and creating additional revenue streams by leveraging data in innovative ways. This approach leads to the generation of new business ideas, the identification of alternative revenue sources, and the development of forward-thinking solutions.
Key success measures:
- The proportion of efforts yielding transformative insights vs. optimization or no insights
- New revenue generated from D&A initiatives
Best Practices for Implementing a Data Analytics Strategy
To get the most out of data analytics, your business needs a clear strategy. But for that strategy to work long-term, you should follow best practices that align with both business goals and evolving technology.
Start small, scale fast: Pilot projects to demonstrate value before full deployment.
Cross-department collaboration: Involve IT, business, and data teams for a holistic approach.
Data democratization: Make data accessible across the organization to foster innovation.
Regular review and adaptation: Continuously refine the strategy based on business and technological changes.
From Data Strategy to Action: Bridging Planning and Operations
For a D&A strategy to succeed, capabilities play a pivotal role in linking the strategic vision to day-to-day operations. Once the “why” and “what” of the strategy are defined, organizations must focus on the “how” by selecting an appropriate operating model and assessing the capabilities needed to execute the strategy.
A thorough capabilities assessment helps identify the current strengths and gaps within the organization. This includes evaluating data management, analytics talent, and data literacy skills. The Gartner IT Score can be used as a self-assessment tool to measure these capabilities. Over 2,000 benchmarks were designed to help CIOs measure, prioritize, and improve function's performance.
The 2022 Gartner CDAO survey revealed poor data literacy as a major barrier to success, highlighting the need for organizations to invest in developing a data-literate workforce to fully leverage data assets and improve return on investment.
Common Pitfalls and How to Avoid Them
When implementing a data analytics strategy, organizations often encounter challenges that can undermine success if not addressed proactively. This section highlights common pitfalls — from unclear objectives to inadequate data governance — and provides practical guidance on avoiding these issues. By understanding and preparing for these potential obstacles, businesses can strengthen their approach, ensuring a strategy that delivers consistent, long-term value.
Pitfalls | How to Avoid |
Lack of Clear Objectives | Start with clear goals and regularly review for alignment |
Underestimating Data Quality | Invest in data governance and quality monitoring |
Ignoring Change Management | Develop a robust change management plan with communication |
Overloading the Strategy | Focus on high-impact initiatives, delivering value incrementally |
Lack of Skilled Personnel | Invest in training or hire skilled data professionals |
Poor Data Governance | Implement clear governance policies around access and security |
Focusing on Technology Over Needs | Ensure technology choices are driven by business needs |
Failure to Iterate and Adapt | Regularly update the strategy to stay aligned with evolving trends |
Your Go-To Data Analytics Strategy Partner
Your business already generates valuable data—now make it work for you. A clear data analytics strategy helps you uncover insights, improve decision-making, and stay competitive as AI reshapes industries.
Collecting data isn’t enough—you need to extract real value with the right approach, one that connects planning to execution and keeps pace with new technologies. Consider professional support for your data and analytics initiatives whenever you want to speed up progress and avoid bottlenecks.