A data-driven approach is now becoming inherent to every sector, from eCommerce to financial services, banking, healthcare, technology, manufacturing, education, life sciences, retail, transportation, entertainment, and more.
Data analytics empowers businesses to maximize the results of data-driven strategies, going beyond extracting historical insights to forecasting future outcomes and enhancing business goal achievement.
Read on to find out how data analytics works and how exactly companies can use it for operational transcendence.
What is data analytics for business? The definition
Data analytics is an intelligence collection process in which gathered raw data is examined, analyzed, and converted into actionable insight aimed at gaining useful results.
Initially, incomprehensible data undergoes processing, sorting, and analysis to become intelligible for a broader audience, beyond just data specialists. Analyzing historical data allows businesses to detect trends, patterns, and underlying causes across all major departments.
Many organizations employ a combination of business analysis software, including statistical tools, predictive modeling apps, and data mining platforms. These tools collectively contribute to enhancing efficiency, boosting revenue, and increasing productivity.
The collected intelligence can further be disseminated to key decision-makers within the organization to guide and positively influence their strategies.
Benefits of data analytics in business
Conducted at a modest or expansive level, effective data analysis holds immense potential to transform business outcomes.
Driving substantial improvements, such as an accelerated logistics process or shipping automation, integrating data analytics enhances operational efficiency, boosts profitability, and provides valuable insights into market dynamics and your customers’ behavior.
Let’s break down the exact ways in which introducing data analytics can help you bring out the best in your company’s endeavors:
Informed decision-making
By leveraging data analysis to guide your business choices you will rely on factual evidence, numerical data, and observed trends.
Nobody doubts your intuition, but with these tools in place, the big picture becomes much clearer.
By relying on this data-driven approach, your company can future-proof its initiatives with robust groundwork, strategic planning, and unshakable policy formulation.
A business plan powered by data analysis ensures the optimal resource allocation and establishes a clear bulls-eye target for goals, objectives, and overall areas that promise the most returns.
Customer segmentation & targeting
It’s undeniable that customers play a pivotal role in every business. Leveraging analytics provides a comprehensive view of all customer-related facets, including their communication channels, demographics, interests, habits, and purchase behavior.
Serving as the tip of your spear, this approach can help you identify untapped customer segments and further enhance your marketing strategy. Additionally, monitoring user reviews and categorizing data on issue resolution gives you the chance to polish up your customer service performance.
Enhanced marketing efforts
A better understanding of your customers grants you the prowess to create a more productive marketing and sales process. Data analytics provides critical intelligence on marketing campaign performance, enabling businesses to optimize strategies for superior outcomes.
Shrunk expenses and optimized costs
Cost reduction stands as another significant advantage. Advanced predictive analytics tools allow businesses to identify improvement opportunities, recognize trends, and discern patterns that are otherwise invisible to the naked eye.
By doing so, you can create a strategic action plan, ultimately saving resources and avoiding misdirected efforts. What’s more, predictive analytics allows organizations to anticipate changes in sales and demand. Having such acute senses can help you augment your production and supply planning.
How it works: Data analytics steps & powerful tools
Systematic data analysis follows a structured path, encompassing multiple essential stages. These steps are pivotal as they guarantee the precision and practicality of the newfound data insights.
Drawing from over 25 years of real experience, our team at Coherent Solutions has been delivering measurable impact on business success for our clients through data and digital product engineering.
We’ll guide you through each phase, starting from defining objectives, culminating in prominent data analytics activities, and concluding with data storytelling.
Step 1: Defining the questions to be answered
Rushing into things unprepared is never a good practice. Before you even start, you need to state your goals and frame clear questions that your analysis aims to address.
This step is essential because it determines the course of the entire process. It requires you to grasp the issue you’re working on, understand its intensity, pinpoint reliable data sources, and set the standards to measure and assess the results of your data analytics process.
Real example: How Airbnb formulates objectives
Airbnb’s products are not as tangible as Apple’s latest Vision Pro creation. They don’t sell physical items but rather the experience of places such as Paris, Ibiza, and even more exotic spots like Bli Bli.
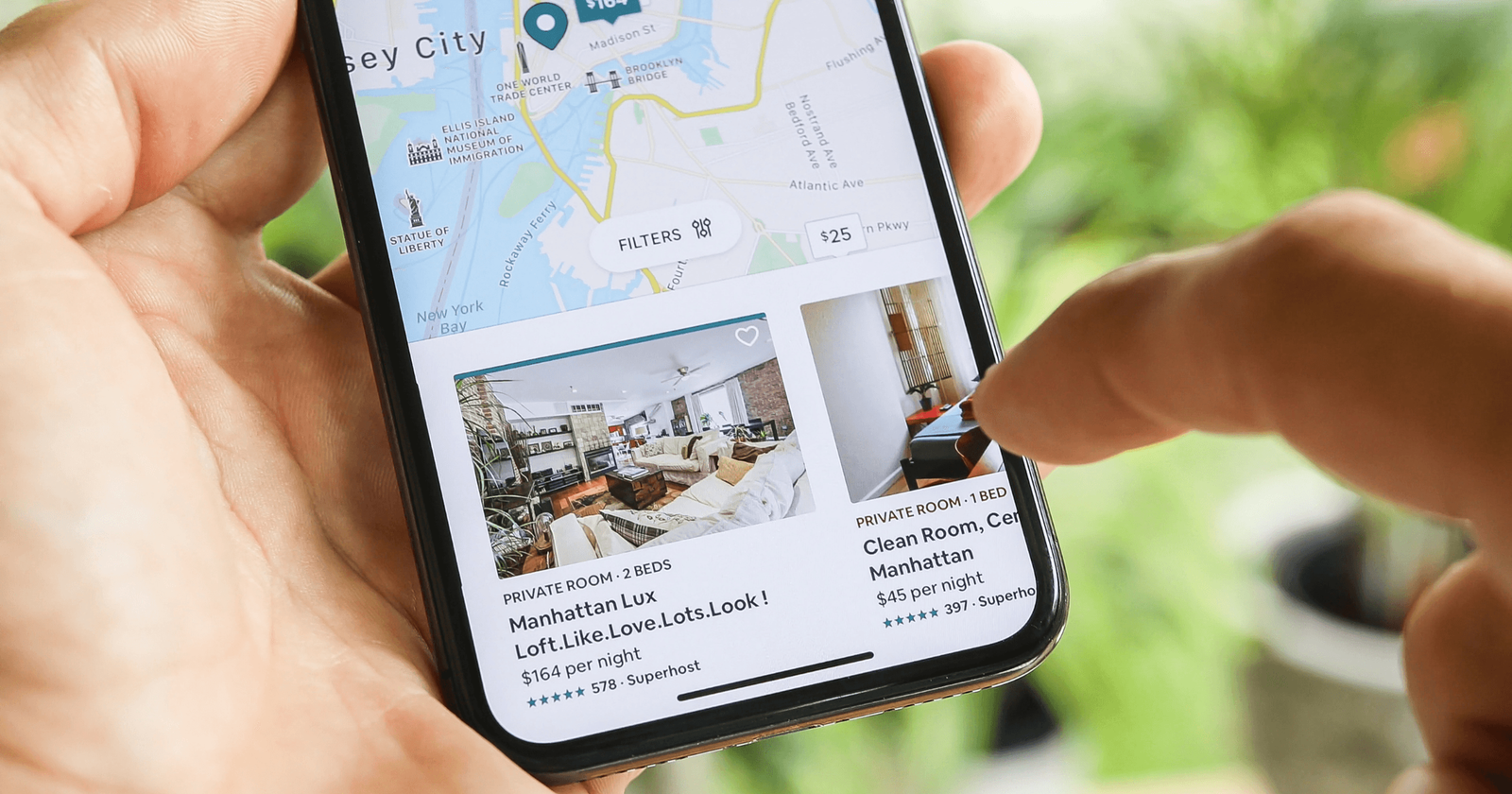
The team’s main aim here is to assist travelers in finding the best accommodation options, enriching their experiences in these hidden travel gems. The true challenge for Airbnb lies in navigating the uncharted terrain of places like these in search of hosts and locations.
They do it by constantly working on improving their search algorithm. With a wealth of data and user experience feedback acquired over the years, and by applying data analytics practices, they’ve made huge progress from the early days of starting up.
Here’s how they’ve defined their goals as the first step in their data analysis process:
Goal: Their goal is to enable their community to provide real-time insights to prospective visitors about exceptional accommodation experiences. Additionally, they aim to apply the same model across various global destinations.
Data sources: A rich dataset comprising guest and host interactions, the location where the person searched, listing quality acquired from Google, and data on users’ behavior.
They set out to measure the data analytics results by assessing how diverse and personalized their search algorithm becomes from the applied data model.
Data analytics tools for setting up objectives
For the most part, your business acumen, professional skills, and creative thinking are all that’s needed to set you on the right track in data analysis.
As for software tools, be on the lookout for KPI dashboards such as Databox, Stashboard, or DashThis. These tools are valuable for creating straightforward dashboards at the outset and conclusion of the data analysis process.
Step 2: Business data collection
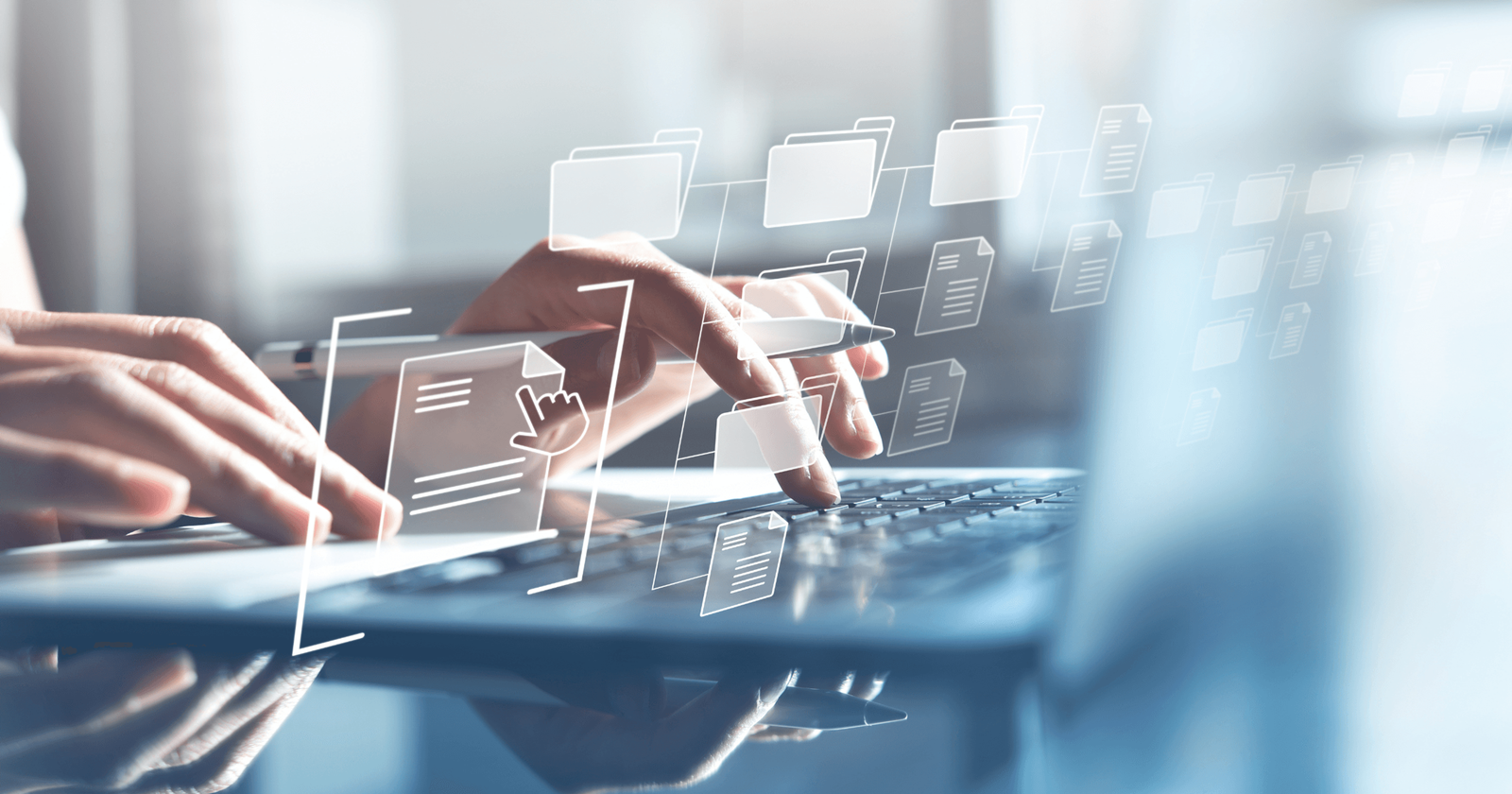
After clarifying your goals and formulating the right questions to answer via data analysis, the next logical step involves gathering a data pool. This is one of the most important steps since the quality of the collected data, in many ways, will define the effectiveness of your data analytics processes.
Data collection can be accomplished using diverse approaches, including surveys, interviews, observations, or extracting information from existing databases.
On a grand scale, there are three types of data sources for you to look at:
- First-party data: Information directly collected from customers by you or your company via customer relationship management systems (CRM) and other tools.
- Second-party data: Secondary data sources obtained from another company, usually via some kind of API functionality or a marketplace. Often very structured and reliable.
- Third-party data: Information that a third-party organization collects and consolidates from various sources. Unstructured and unorganized information gathered in various forms (textual, audio, visual, etc).
Data analytics tools for data collection
For the most part, all you will need for aggregating and refining your datasets is an enterprise Data Management Platform (DMP) like Oracle, Salesforce, or SAS.
In some cases, you will also need an integration platform like Integrate.io or modern and more flexible platforms such as Pimcore or Talend Data Fabric.
Step 3: Data cleansing and preparing for analysis
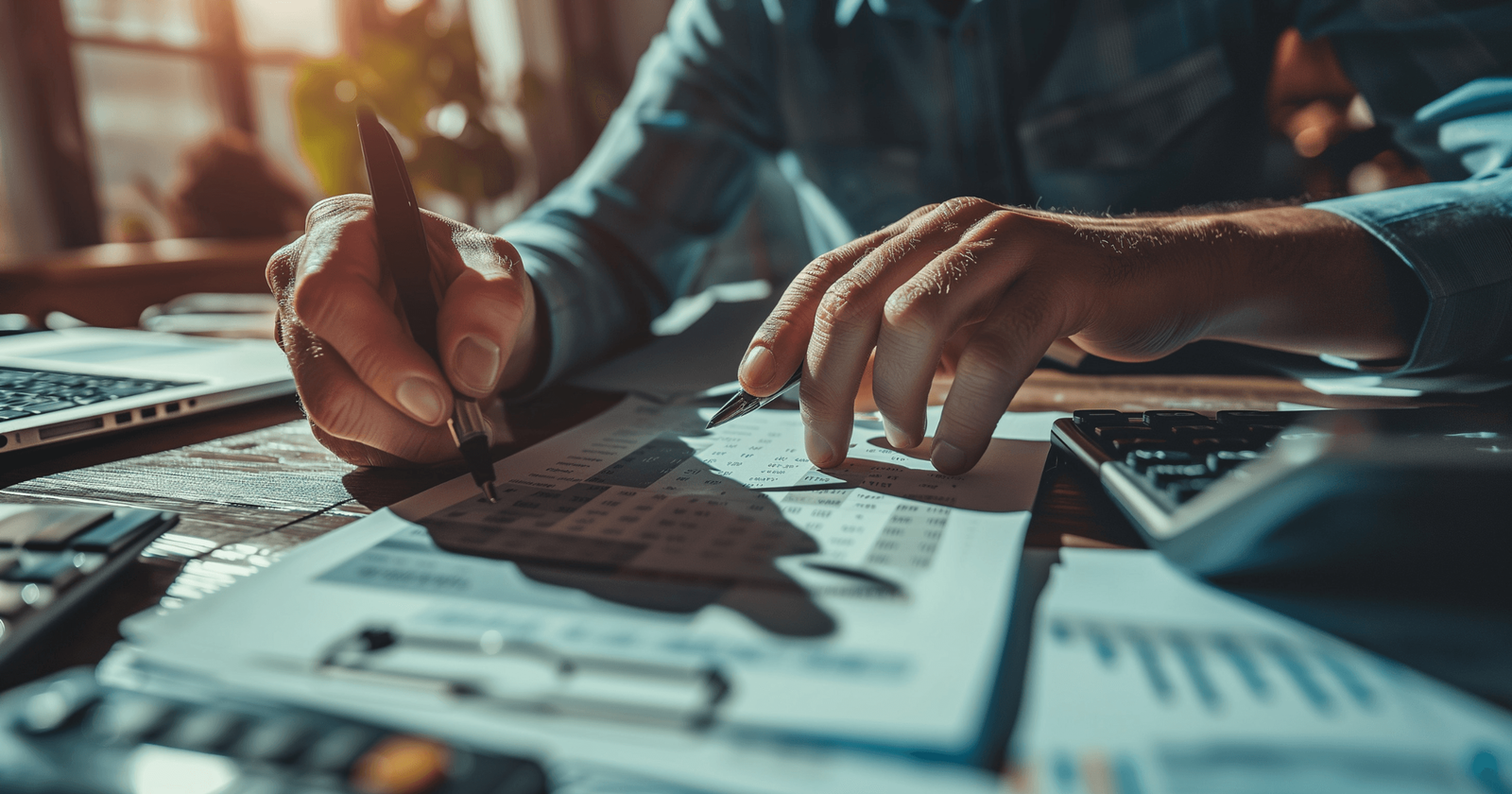
After you’ve collected enough data, the next steps involve its preparation for analysis. Raw and unpolished data negates the effects of data analytics. Data cleaning or ‘scrubbing,’ is essential to ensure that you reach the goals you set during the first step.
Here’s what you need to accomplish:
- Fixing structural errors: Data may be presented in different forms or have inconsistencies, so aligning it with a uniform format will be one of your objectives during cleaning.
- Handling outliers: You will need to filter out data points that deviate significantly from the norm.
- Removing duplicate or irrelevant observations: Don’t forget to eliminate unwanted data points and enrich your data pool with diverse information, so you can reach your business data analysis goals much faster.
- Filling in the gaps: While organizing your data, you may encounter significant gaps where essential information is missing. Once you’ve pinpointed these gaps, you can proceed to fill them in.
Real example: How Airbnb cleans its data
At some point, the Airbnb team realized their datasets and models were insufficient, as biases led to gravitational pull toward big cities in their search algorithm.
For example, a search for a smaller location, such as the nearby surf town Pacifica, would return some listings in Pacifica and then many more in San Francisco.
To counter this flaw, they eventually normalized data points by the number of listings in the search area. Normalization is a typical technique in data cleansing that involves structuring the data into a series of so-called normal forms to reduce data redundancy and improve data integrity.
Another example: Preparing data for AI healthcare diagnosis
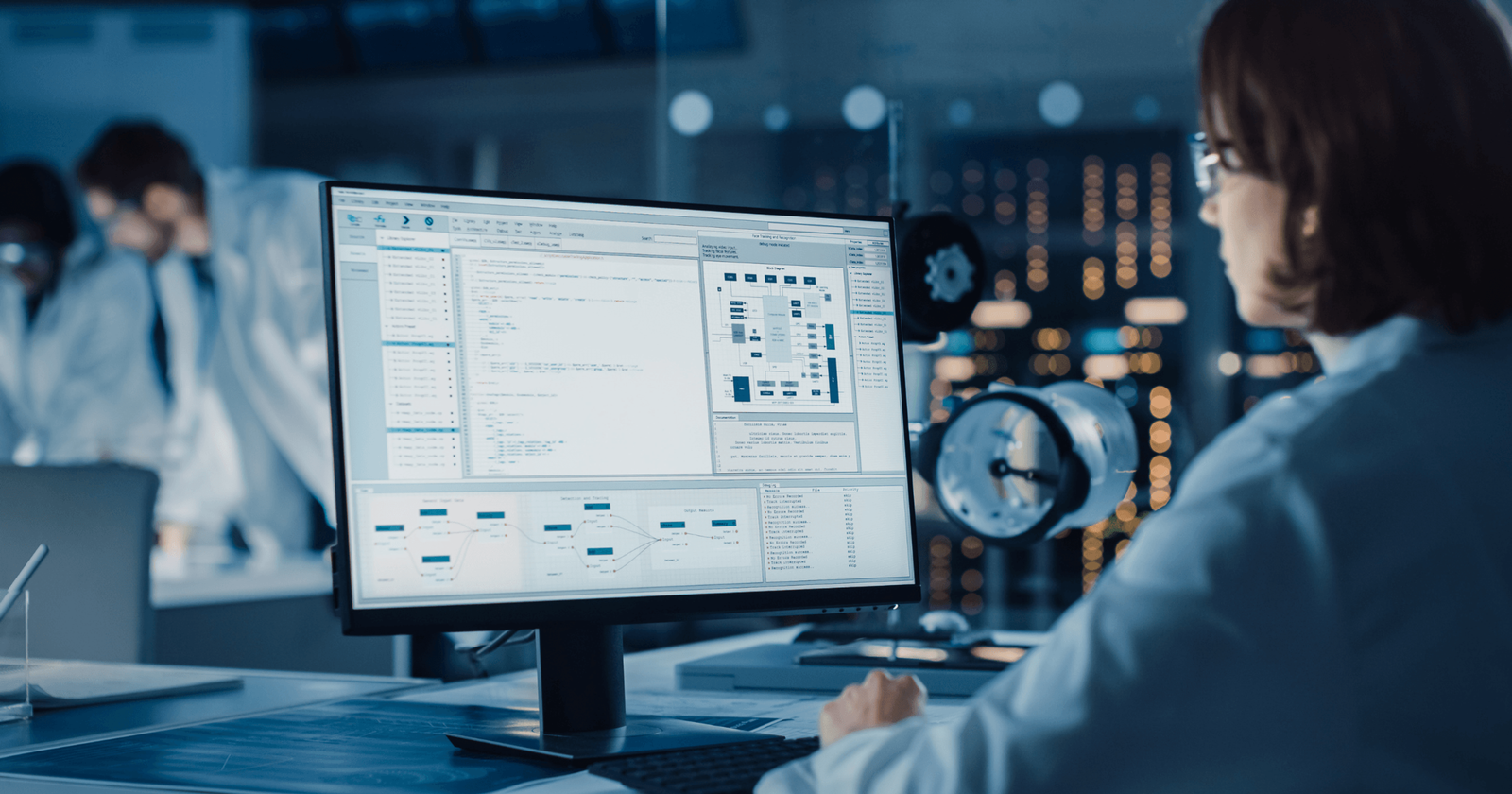
Since Airbnb’s example may seem somewhat less encompassing than needed to illustrate all the ways data sets can be optimized, let’s look at a more vivid case.
An AI ophthalmology startup aimed to establish a digital repository of pathological cases, providing essential guidance and support to aspiring ophthalmologists. Their AI was to be trained on numerous retinal snapshots showcasing a variety of human retina issues.
These snapshots were taken with varying quality cameras, in different lighting conditions, from various angles and distances, and often had unwanted visual artifacts. This visual data collection had to be cleansed and transfigured into a unified format.
The team decided to use an RGBA image format to unify all data and used the following transformation techniques:
- Affine transformations (image rotations, flips, skews, shifts, etc.);
- Quality and color changes (image blurring, contrast, hue, saturation, etc.).
These techniques allowed them to create a dataset that successfully trained an AI model for human retina disease diagnosis.
Data analytics tools to help you clean your data
Open-source options like Easy Data Transform and OpenRefine are great for fundamental data cleaning and exploration. However, they may have serious limitations when dealing with very large datasets.
For more robust data scrubbing, consider using data quality and matching engines like Data Ladder or WinPure Clean & Match.
Step 4: Business data analysis
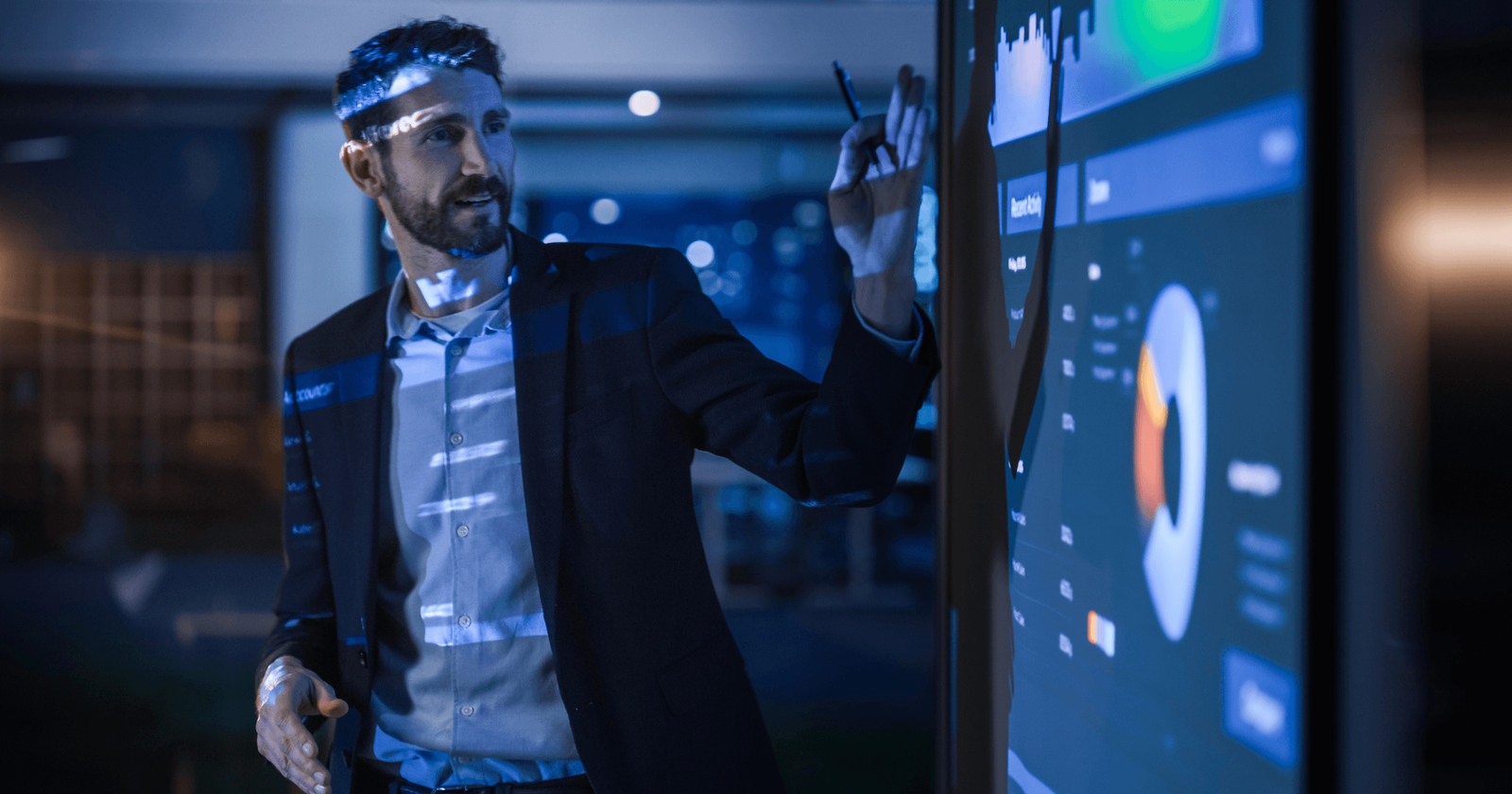
The crux of the data analytics process is, of course, the actual data analysis. This is where statistical and mathematical techniques are used to uncover patterns, relationships, or trends within the data.
The choice of data analysis techniques depends on the objectives you’ve set. There are numerous methods available, including univariate or bivariate analysis, time-series analysis, and regression analysis.
Ultimately, there are four main categories of data analysis:
- Descriptive analysis provides insights into what has already happened. For instance, you might want to measure your ROI over the last five years.
- Diagnostic analysis gives you an idea as to why something has happened. For example, an eCommerce business would want to know why they are witnessing a dire cart abandonment problem.
- Predictive analysis leverages historical data to anticipate the future. In retail, transaction data helps predict trends and seasonal buying patterns, shaping strategic decisions.
- Prescriptive analysis provides recipes for success and recommendations for the future. For instance, data analytics can predict which products are likely to be in demand next winter and help you align your production and distribution strategy accordingly.
We’ll dive deeper into these categories a bit later to make sure you have the best understanding of the possibilities data analysis brings to the table.
Real example: How Airbnb used data analysis to improve their service
To further enhance their search algorithm and eliminate inconsistencies (like the famous ‘mushroom dome’ location appearing in unrelated searches), the Airbnb team made a pivotal decision: they anchored their location relevance model entirely on user behavior data. This strategic shift allowed them to dynamically guide future guests toward exceptional experiences, consistently across global destinations.
Recognizing that hosts often favor requests that align with their availability, the team delved into the intricacies of host behavior. They meticulously examined prior acceptance and decline patterns, as well as trip-specific details. Rather than inundating the algorithm with excessive complexity, they devised a custom set of data filters, applying them in the following sequence:
Accommodation Requests -> Host Preferences -> Preference Coefficients
The outcome was remarkable: a nearly 4% boost in booking conversion rates and a substantial increase in successful guest-host matches. It’s a win-win scenario for all parties involved.
Business data analytics tools for extracting insight
You don’t need to be a guru in Python, R, or SQL to master data analysis as there are plenty of point-and-click tools available. Try the powerful Microsoft Power BI or SAS Business Intelligence’s suite of self-service analytics.
For a more streamlined approach, use Metabase. It’s very popular for its user-friendly and straightforward interface.
Step 5: Data interpretation and visualization
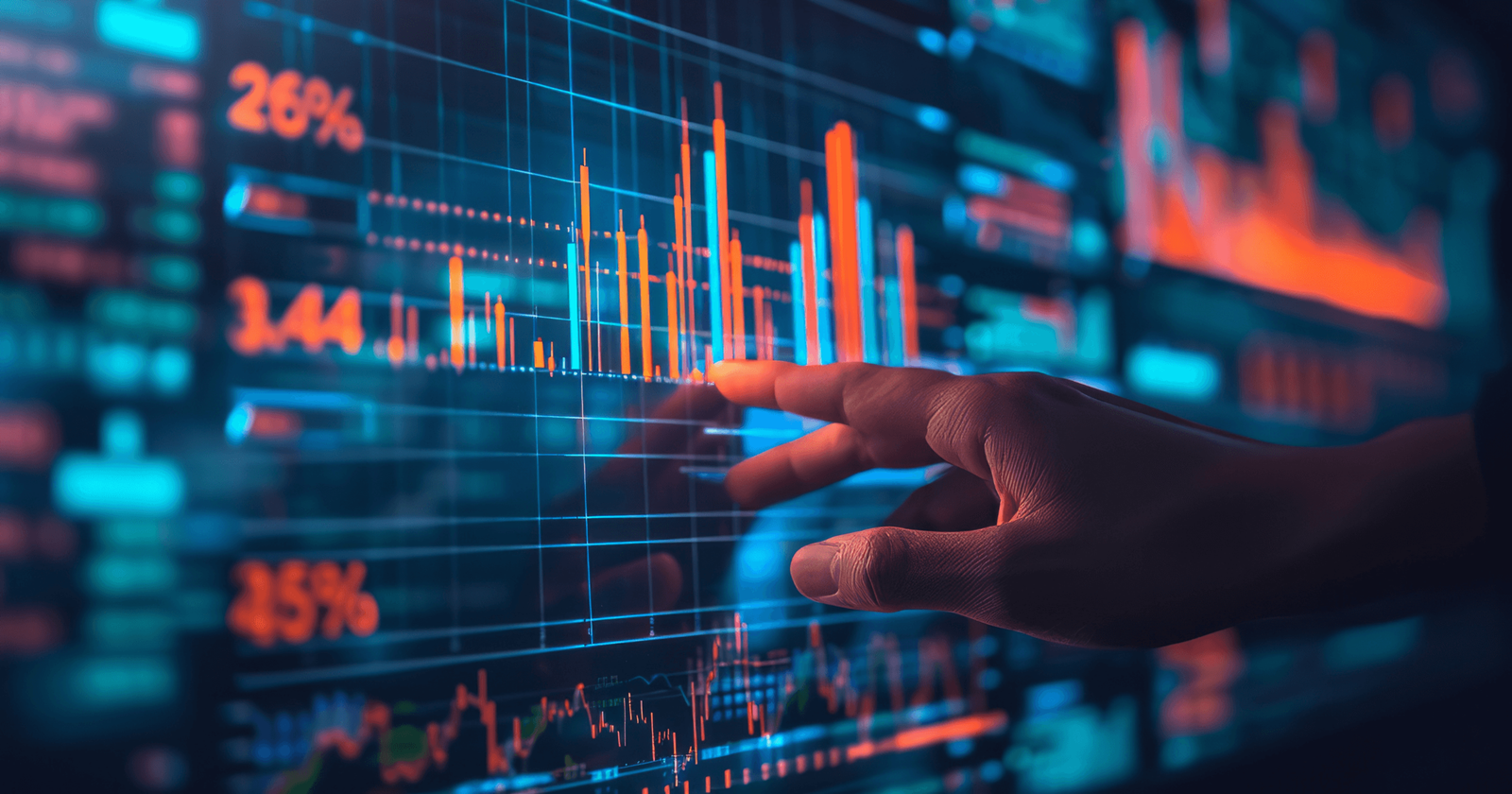
Concluding the data analysis process, we arrive at data storytelling. This entails conveying analysis findings in an engaging and easily comprehensible narrative. Data storytelling is the key to communicating results to non-technical audiences and inspiring data-driven decision-making.
In this step, you might create charts, graphs, or other visual depictions of the data. Visualization plays a crucial role in simplifying complex information, offering a clear and concise view of the findings.
The way you interpret and present results often defines the business’s development trajectory. Based on the data analytics report, your company may choose to restructure, bet on a high-risk product, or even shut down an entire division.
Therefore, it’s crucial to provide your shareholders with a vivid description of the gathered information.
Real example: How presenting data findings to engineers helped Airbnb improve conversion
In 2014, Airbnb leveraged data analytics to customize their search experience based on demographics. They observed that users from specific Asian countries often had a high bounce rate when visiting the homepage.
Upon deeper analysis, they found that these users would click the ‘Neighborhood’ link, browse photos, and then abandon the booking process.
The insights were presented to the engineering team, and a revised interface version was developed specifically for Asian demographics. The updated version featured information about the top travel destinations in China, Japan, Korea, and Singapore.
As a result of these changes, they observed a 10% increase in conversions in these locations.
Data analytics tools for visual storytelling
There’s a variety of data visualization tools, each suited to different business cases and scenarios, including Google Looker Studio, Tableau, and Sisense.
Regardless of the data visualization tools you select, don’t forget to hone your presentation skills as well. Bear in mind that while visualization is powerful, effective communication remains paramount!
Types of data analysis
Now let’s explore the gist of it and dive into the various methods and techniques used in data analytics. As we’ve mentioned before, the four main types of data analysis are: Descriptive, Diagnostic, Predictive, and Prescriptive.
Let’s take a closer look at each of these to assess their value for business and derive the advantages you gain by integrating them into your development funnels.
Descriptive analytics
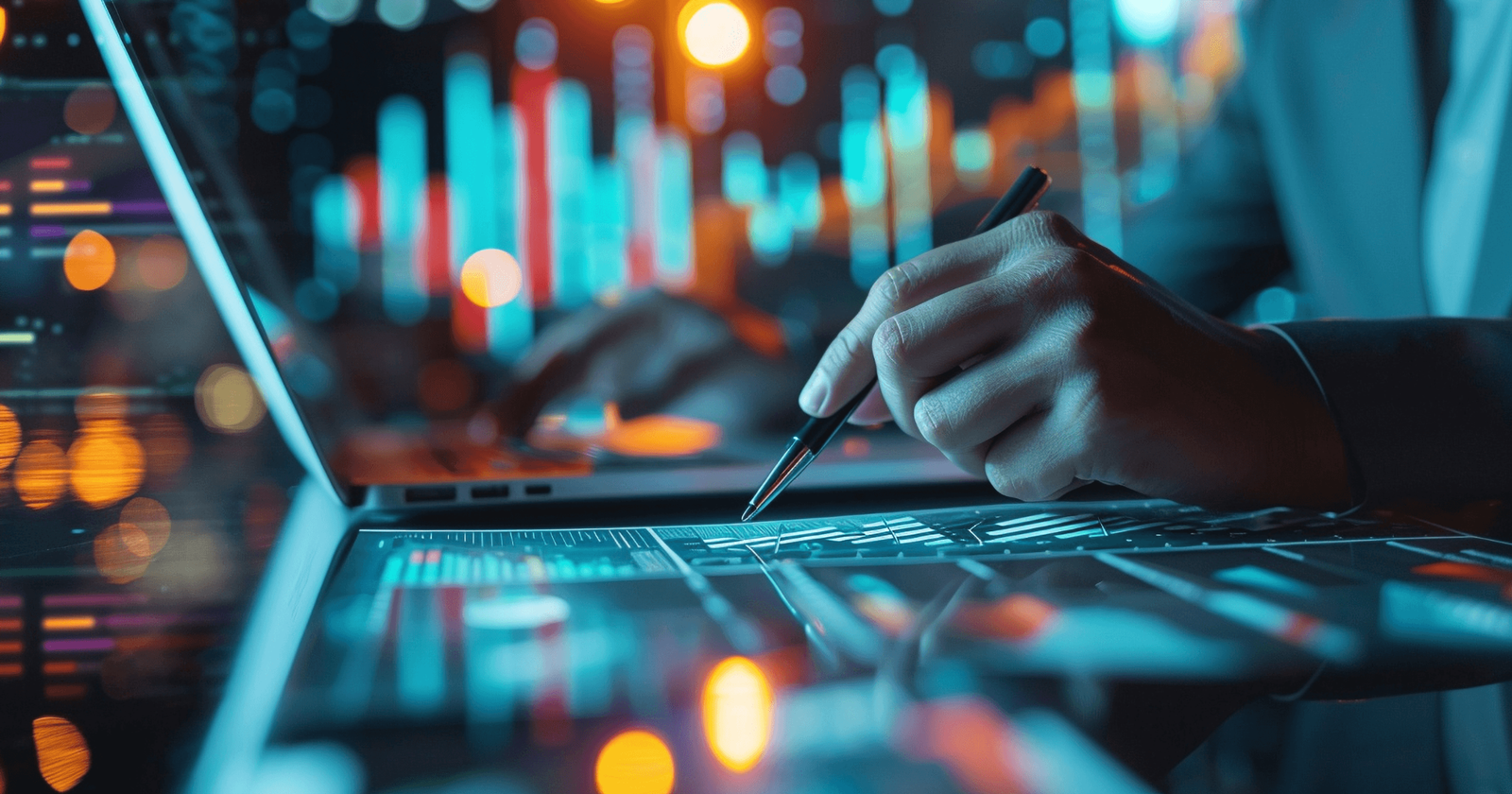
Descriptive analytics stands as the most frequently employed and straightforward type of data analysis. Its primary objective is to summarize large datasets into concise business reports that are clear and comprehensible for business executives.
Descriptive analytics is mainly used on historical data. A great example here would be using it for tracking and managing key performance indicators (KPIs).
For instance, a startup can employ descriptive analytics to monitor their benchmarks for the upcoming funding campaign, such as daily active users, monthly recurring revenue, customer lifetime value, etc.
By aggregating and analyzing this data, and visualizing it, they can present a vivid picture of success to their investors and secure an influx of capital.
Diagnostic analytics
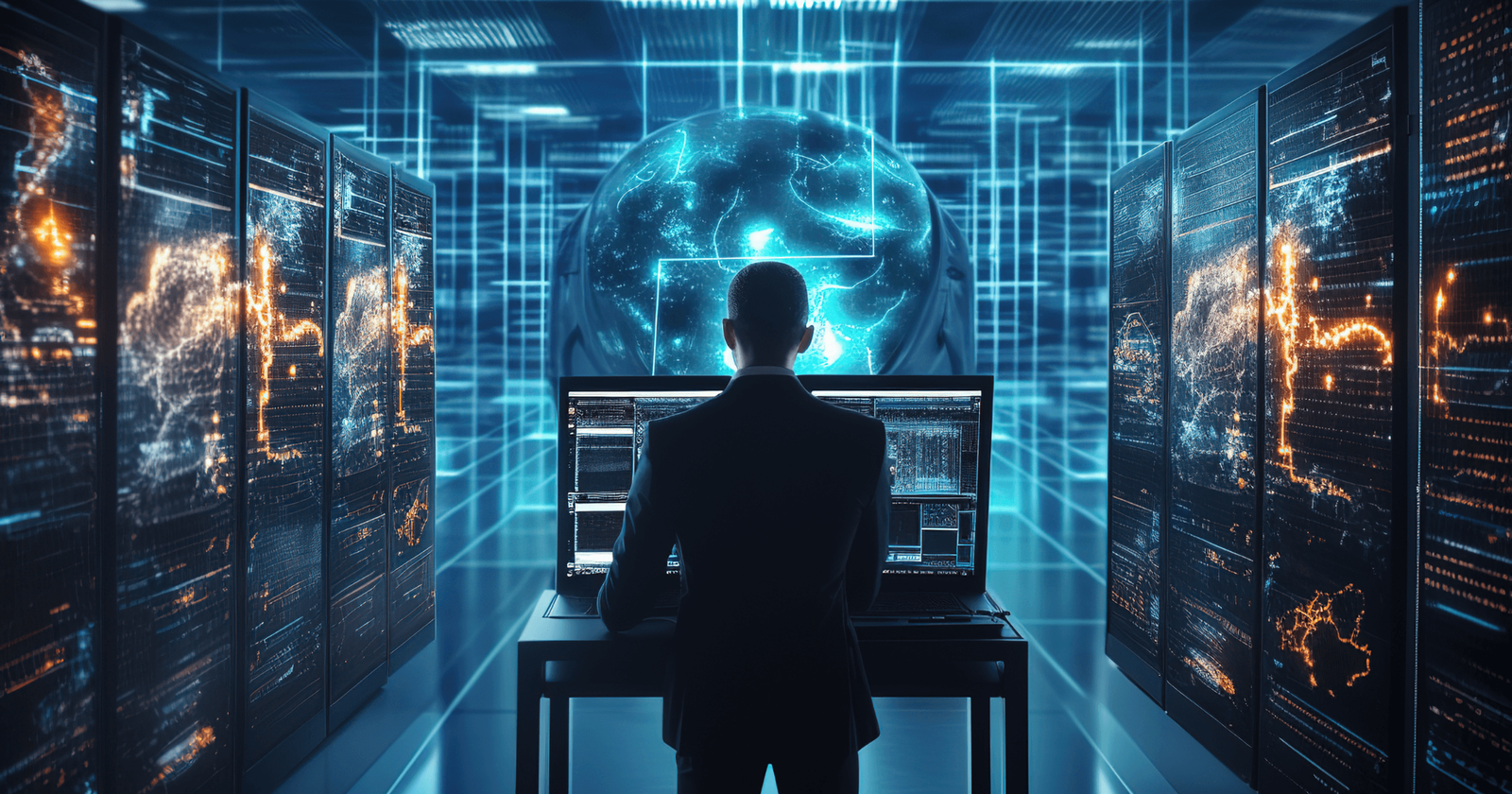
Diagnostic analytics is the most intricate type of data analysis where complex data models and techniques coincide to answer the question of ‘Why?’. Why is there a certain downward or upward trend? Or why is there such low demand for your premium plans?
These analytics methods are used to deep-dive into your pool of data, providing answers to these questions and extracting valuable insights that inspire your next move in product development.
One of the most useful cases for diagnostic analytics is figuring out the motives behind customer behavior.
Let’s imagine the team behind a popular event-driven social media app spots a spontaneous surge in daily users for January. But come February, they fail to repeat the feat.
By conducting diagnostic analysis and using advanced data analytics tools, they can identify various trends across different cultures, demographics, etc., and correlate this data with their app’s latest usage statistics to bring out dependencies and visualize them into useful reports.
For instance, it might turn out that January was big on music festivals, and the results of the analysis showed that most app users are avid festival-goers. The insight could have inspired the team to develop a strategy with more focus on engaging this particular type of audience.
Predictive analytics
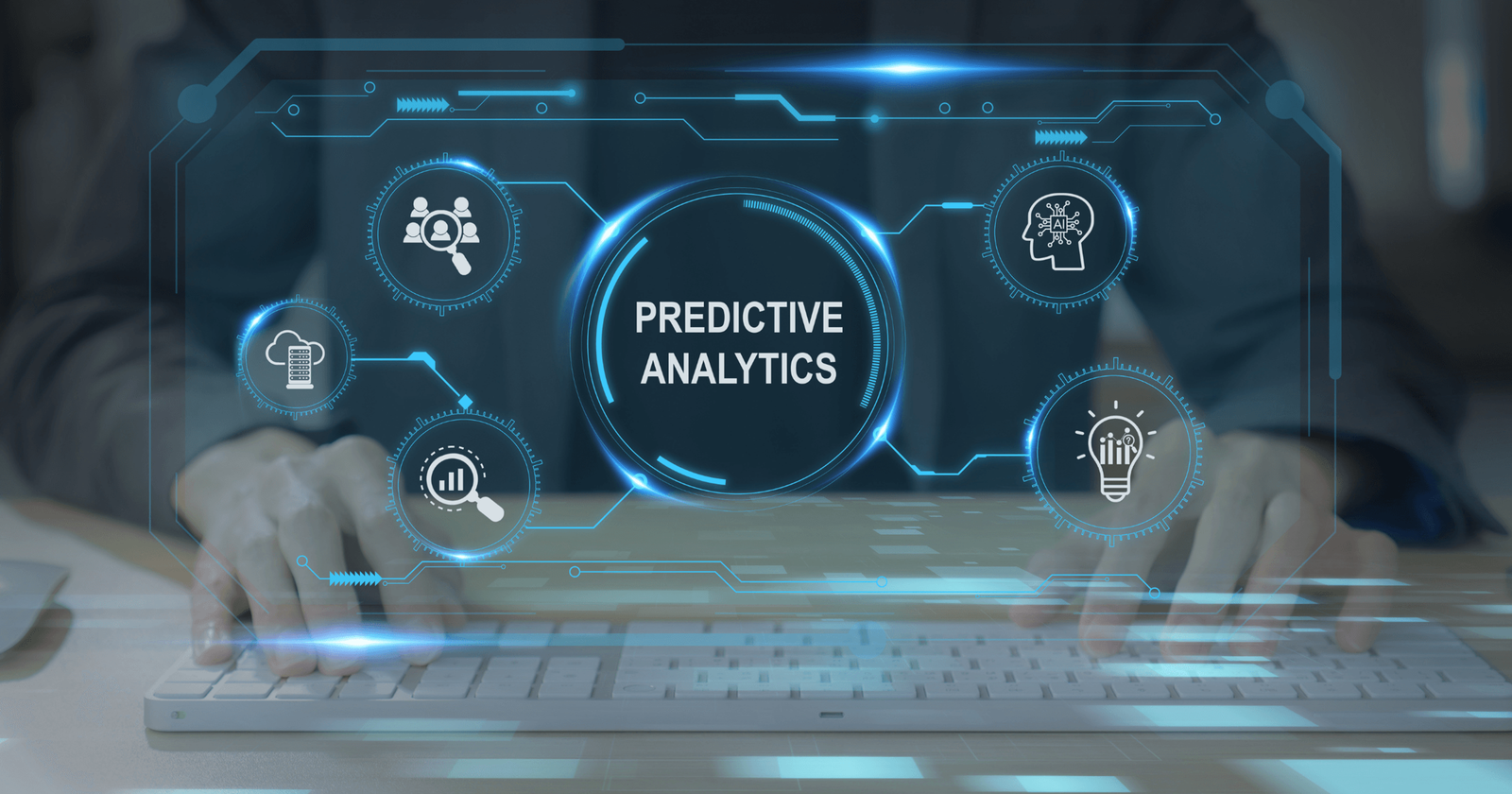
Predictive analysis leverages both current and historical data to enable businesses to forecast future outcomes and performance. It gives them a definitive head start for introducing strategies that anticipate change.
When it comes to marketing, predictive analytics gives birth to actionable reports and propositions on prosperous marketing approaches or new opportunities. The predictions can be based on the performance of earlier campaigns and current market trends.
Another effective use case here is in supply chain management. Supply chain analytics plays a crucial role in predicting and overseeing inventory levels and pricing strategies. This proactive approach can be used to monitor and forecast future performance, demand, and potential disruptions.
It enables businesses to enhance their decision-making, successfully identify and tackle risks, and optimize resource management. Most tempting for businesses is the ability to anticipate the supply and demand at any given time and assess the likelihood of shortages.
Prescriptive analytics
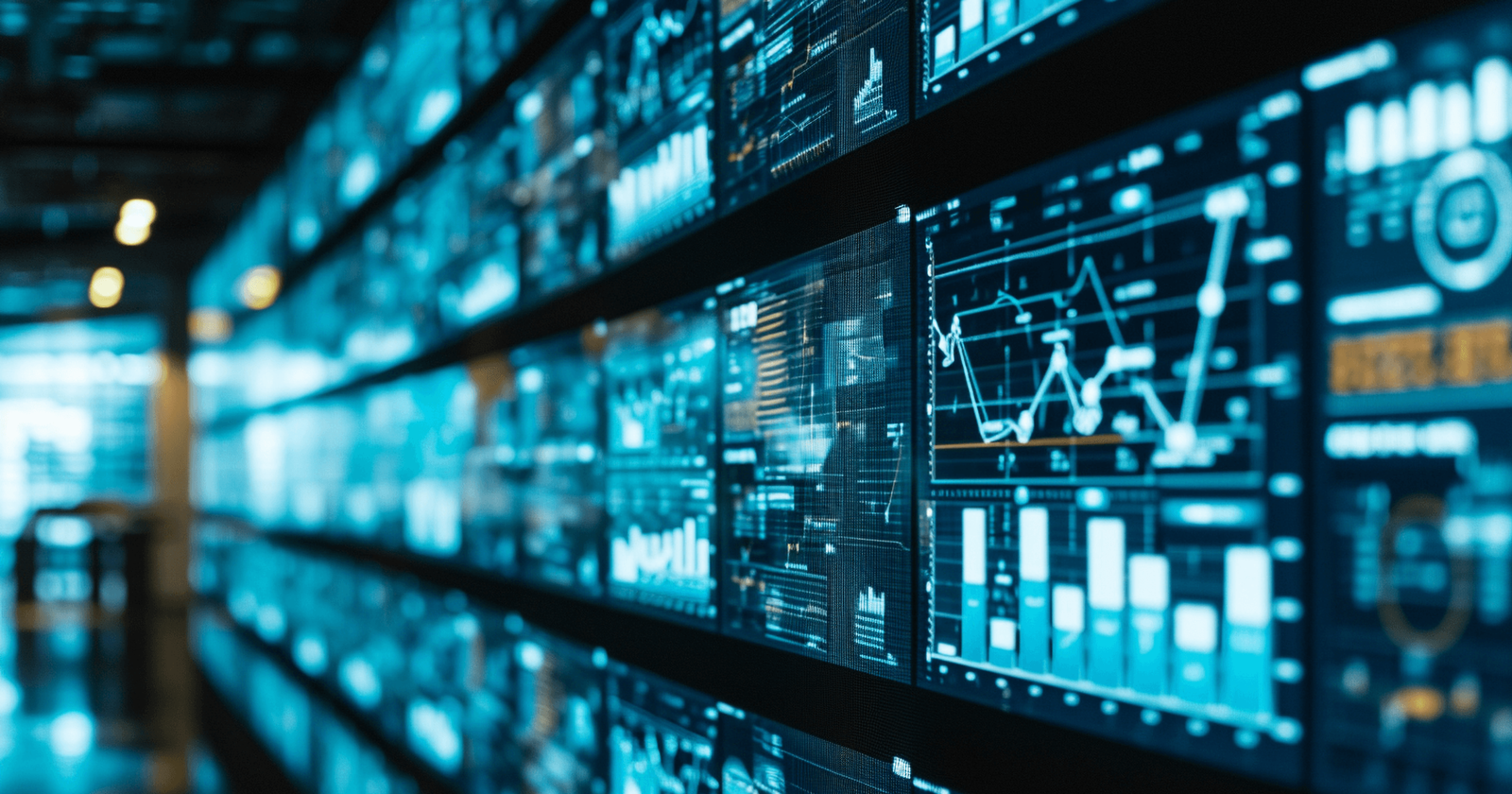
Prescriptive analysis represents the final stage of the data analytics process, going beyond mere prediction. It merges historical and current data along with predictive forecasts.
Studying the provided expansive pool of data, the analytics engine, in most cases powered by modern AI technologies, recommends the best course of action.
For instance, in manufacturing, prescriptive analytics can predict when machinery or equipment is likely to fail. By analyzing historical data and performance metrics, it recommends maintenance schedules, extending lifespan, and minimizing downtime.
Financial institutions use prescriptive analytics to counter fraud. Analytics tools monitor transaction data, detect anomalies, and recommend security measures.
In modern marketing, prescriptive analytics stands at the helm of campaign strategies.
There’s a variety of ways prescriptive analytics tools can be used to maximize productivity and top-up your revenue.
Conclusion
In business, making intuitive decisions is a gamble, and leaving it all to chance is a luxury no company can afford. Data-driven strategies provide managers and executives with the power of X-ray vision when it comes to spotting productivity gaps, creating opportunities, and making impactful decisions.
By embracing data analytics, your business will become more agile, resilient, and responsive to rapid market changes. Constantly learning from a vast data landscape in today’s dynamic world, a business sets itself up for continuous evolution.
It’s a long-term winning strategy that creates an innovative corporate culture and gives you a competitive advantage in the market.
Coherent Solutions is your trusted data analytics partner
Creating a data analytics pipeline from scratch may prove to be a tricky endeavor. Out-of-the-box open-source solutions are sometimes very limited in functionality and enterprise platforms are pricey and never provide complete flexibility to specific business cases.
Oftentimes, you just can’t go around the necessity for technical expertise. Experienced data scientists, analysts, and developers, like the ones we have at Coherent, know the ins and outs of building and integrating data-driven models into established and running business processes.
Acting as your trusted partner, our team can harness the flexibility of data science development tools like Python, R, and SQL to build the entire data analytics infrastructure tailored to your business. Taking a shorter route, we can also customize enterprise platforms like Salesforce, Microsoft Power BI, and others to meet your individual needs and preferences.
Discover how our data analytics services can elevate your business strategy to new heights. Contact us now to discuss the specifics of your data-driven business goals, and let’s embark on a journey of transforming your raw data into actionable insights.
FAQ
1. What does data analytics in business do?
Businesses utilize data analytics to uncover crucial growth prospects, identify emerging market trends, analyze and predict customer behavior, guide product development, optimize marketing efforts, improve operational efficiency, manage risk, and inform strategic and tactical decisions across various departments.
2. What are the 4 types of business analytics?
Descriptive, diagnostic, predictive, and prescriptive analytics are the 4 types of business analytics that assist businesses in maximizing the value of their data.
3. Why is business analytics important?
Business analytics serves as an essential tool for companies aiming to excel in today’s data-driven, dynamic business landscape. By predicting future trends and outcomes, it empowers proactive decision-making and effective risk management.
4. What are the 5 steps in data analytics?
The data analysis process involves five key steps:
- Business problem identification: Start by identifying the relevant business questions or objectives that need data-driven answers.
- Data collection and storage: Gather and store the necessary data from various sources, ensuring its quality and reliability.
- Data cleaning and preparation: Cleanse and prepare the data to remove inconsistencies, missing values, and errors.
- Data analysis: Perform in-depth analysis using statistical methods, machine learning, or other techniques to extract insights.
- Data visualization and communication: Present the findings visually through charts, graphs, or reports to empower decision-making and communication.