This article delves into the dynamic AI trends transforming the pharma and biotech sectors in 2024-2025 and beyond. We’ll explore technological breakthroughs, market growth, and the emerging opportunities that promise to redefine the future of healthcare and drug development.
The pharmaceutical industry is on the brink of a major transformation, driven by the power of AI. From streamlining drug discovery to accelerating development and approval processes, AI is unlocking billions in value. According to BioPharmaTrend, AI is projected to generate between $350 billion and $410 billion annually for the pharmaceutical sector by 2025, driven by innovations in drug development, clinical trials, precision medicine, and commercial operations.
The Current State of AI Adoption in Pharma and Biotech
Let’s explore the role AI is playing in the pharma and biotech sectors, observe relevant statistics and identify key players in the market.
Adoption of AI Technologies in Biopharma
Can artificial intelligence truly reshape the way medicines are developed and delivered? The numbers suggest it already is. By 2025, AI spending in the pharmaceutical industry is expected to hit $3 billion, reflecting a surge in its adoption to reduce the hefty time and costs associated with drug development. Alliances focused on AI-driven drug discovery have skyrocketed—from just 10 collaborations in 2015 to 105 by 2021—demonstrating its growing influence.
Leading the pack are ‘AI-first’ biotech firms, where AI isn’t just an add-on but the backbone. A 2023 Statista survey reveals that 75% of these trailblazers heavily integrate AI into drug discovery. However, traditional pharma and biotech companies lag behind, with adoption levels five times lower.
Why the slow pace for some? It’s not just about technology but transforming the way business is done. According to PwC, 53% of pharma finance leaders are prioritizing AI and advanced analytics to automate processes and drive efficiency. The goal is bold: to rethink operations from the ground up.
Whether moving cautiously or charging ahead, the adoption of AI is no longer optional. It’s the key to unlocking innovation, and the companies leading the charge are those daring to invest in the future today.
Major Players in the Pharmaceuticals Industry
AI is becoming a driving force in the pharmaceutical industry. The global AI in pharmaceutical market is estimated at $1.94 billion in 2025 and is forecasted to reach around $16.49 billion by 2034, accelerating at a CAGR of 27% from 2025 to 2034. And leading companies are seizing its potential to transform research and development.
Pfizer
Pfizer has embraced AI through partnerships with Tempus, CytoReason, and Gero, integrating it into drug discovery, clinical trials, and patient population analysis. A standout achievement? Accelerating the development of COVID-19 treatments, including Paxlovid, with AI’s help.
AstraZeneca
With collaborations like BenevolentAI and Qure.ai, AstraZeneca employs AI in developing treatments for chronic kidney disease and pulmonary fibrosis. AI also plays a pivotal role in enhancing drug discovery and optimizing clinical trial designs.
BenevolentAI
This UK-based innovator specializes in AI-powered drug discovery, focusing on selecting precise drug targets. Their partnerships with AstraZeneca and Novartis highlight their expertise in advancing pharmaceutical research.
Janssen (Johnson & Johnson)
Janssen is leading the charge with over 100 AI projects in clinical trials, patient recruitment, and drug discovery. Their Trials360.ai platform exemplifies how AI can streamline trial processes, improving speed and efficiency.
Insilico Medicine
Renowned for its cutting-edge use of AI, Insilico Medicine combines deep learning models with drug design and synthesis, making significant strides in accelerating drug discovery.
Roche
Topping the Statista AI readiness index in 2023, Roche is setting the standard for AI adoption in pharma. Its success stems not only from in-house talent and innovation but also strategic acquisitions of smaller, tech-driven firms to bolster its capabilities.
The Impact of AI on Drug Discovery and Design
The traditional path to developing a new drug is long, costly, and often uncertain. On average, it takes 14.6 years and around $2.6 billion to bring a new drug to market. But what if artificial intelligence could dramatically shorten this journey? AI is already reshaping drug discovery and design by facilitating key stages and making the entire process more efficient, cost-effective, and successful.
At the heart of this transformation is target identification. Traditionally, pinpointing novel drug targets involves painstaking trial and error, but AI can sift through vast amounts of biological data to uncover potential targets that might otherwise go unnoticed. Such an approach allows researchers to zero in on the most promising opportunities faster and accelerate the drug development process.
AI doesn’t stop there. Once targets are identified, it can evaluate drug-target interactions and analyze disease mechanisms with a level of precision that was previously impossible. By understanding how diseases progress at a molecular level, AI aids designing more effective drugs, ensuring that compounds are not only innovative but also highly specific to the target. AI enables quicker adjustments to chemical structures and increase the chances of success. By 2025, it's estimated that 30% of new drugs will be discovered using AI, marking a significant shift in the drug discovery process.
Here’s where it gets interesting: AI-enabled workflows have been shown to reduce the time and cost of bringing a new molecule to the preclinical candidate stage. For complex targets, these efficiencies could save up to 40% of time and 30% of costs. In a field where the stakes are high, these savings can be a game-changer, allowing companies to test more compounds and find breakthroughs faster.
Perhaps most notably, AI has the potential to increase the probability of clinical success. Traditional drug development sees only about 10% of candidates making it through clinical trials. AI-driven methods, however, are poised to change this. By analyzing large datasets and identifying promising drug candidates earlier in the process, AI increases the likelihood of clinical success, making drug development not just faster, but smarter.
AI’s Role in Optimizing Clinical Trials in Biopharma
AI is transforming clinical trials in biopharma, turning traditional inefficiencies into opportunities for innovation. But what does this transformation really look like in action, and what outcomes can the industry expect?
Patient Recruitment
Recruiting the right patients has always been a major challenge in clinical trials. But AI is here to streamline the process. Traditionally, patient recruitment involves manual searches through patient databases, a time-consuming and error-prone task. With AI, machine learning models analyze vast amounts of Electronic Health Records (EHRs), identifying eligible participants quickly and with high accuracy. TrialGPT, for example, automates this process by matching patients to suitable trials based on their medical histories and trial criteria. This not only speeds up recruitment but also helps ensure greater diversity in trials and even predicts patient dropouts, preventing trial disruptions. This faster and smarter recruitment process can significantly cut down trial delays.
Trial Design
Designing clinical trials used to involve a lot of guesswork and rigid parameters. AI is changing that by making trial design more dynamic and patient-focused. Using real-world data (RWD), AI algorithms can identify subgroups of patients who are more likely to respond positively to treatments. This means trials can be adjusted in real-time to reflect patients’ responses, optimizing for better results. AI also helps refine inclusion criteria to exclude likely non-responders, cutting down the trial duration by up to 10% without compromising the integrity of the data. The result is more efficient, targeted trials that bring drugs to market faster and more accurately.
Data Analysis
AI’s role in data analysis during trials is nothing short of groundbreaking. In traditional trials, researchers often wait until the end of the study to analyze results, but with AI, real-time data analysis is now possible. AI continuously processes data from patients throughout the trial, identifying emerging trends, predicting outcomes, and even adjusting trial protocols on the fly. This dynamic data analysis helps optimize the trial, reduces the likelihood of errors, and ultimately saves resources. Moreover, Generative AI can predict the success rate of a trial by analyzing historical data, increasing the probability of success and cutting costs. With AI’s involvement, pharma companies can expect up to $25 billion in savings in clinical development alone.
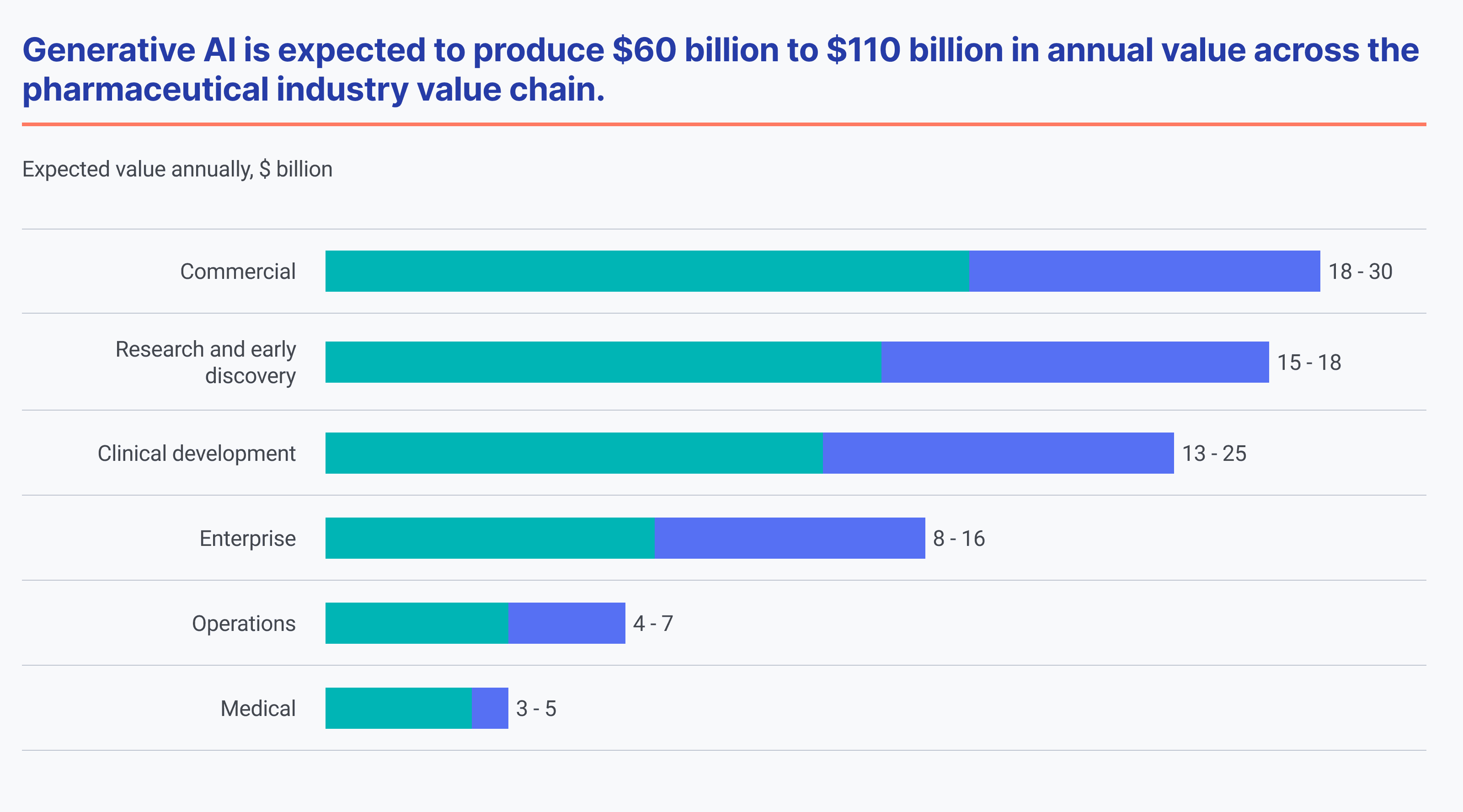
AI in Pharmaceuticals Supply Chain
AI technologies are changing the pharmaceutical supply chain management in ways you might not expect. From manufacturing optimization to real-time supply chain management, these advancements are driving efficiency and reliability. Let’s discover what exactly makes these innovations so impactful.
Manufacturing Optimization
AI-driven systems are optimizing pharmaceutical manufacturing by reducing errors and improving product consistency. Real-time analytics allow production lines to adjust dynamically, enhancing efficiency and quality. By integrating smart devices, AI powers Industry 4.0 with robotics and IoT for better control, leading to smoother operations and faster production cycles.
Predictive Maintenance
In pharmaceutical production, unexpected machine failure can cause costly delays. AI-powered predictive maintenance identifies issues before they happen by analyzing sensor data from equipment. This proactive approach helps avoid downtime, keeping production running smoothly and maximizing efficiency and uptime.
Supply Chain Management
AI is enhancing supply chain management by improving demand forecasting and inventory optimization. With predictive analytics, pharmaceutical companies can minimize waste and ensure timely deliveries. Real-time tracking of shipments enhances visibility, while AI monitors storage conditions to maintain product integrity, ensuring that drugs are always delivered safely and on time.
AI in Biotechnology and Pharma Trends for 2025
We all know that artificial intelligence and related technologies are making waves. Now, we’ll have a closer look at the major trends of AI in the biotechnology and pharma industries for the next decade.
Pharmaceutical AI Market Continues to Grow
The market for AI in pharma and biotech is experiencing rapid growth, signaling a promising future for the industry. With an increasing reliance on AI to drive innovation and efficiency, the sector is poised for significant expansion in the coming years.
In 2023, the AI in the pharma market was valued at $1.8 billion. By 2034, this figure is expected to soar to $13.1 billion, reflecting a robust compound annual growth rate (CAGR) of 18.8% from 2024 to 2034.
The global market for AI in drug discovery alone is projected to increase from $1.5 billion to approximately $13 billion by 2032. Additionally, AI-based solutions in clinical research are forecasted to exceed $7 billion by the end of this decade, underscoring the growing demand for AI-driven advancements across the entire pharmaceutical value chain.
Rise of AI-Driven Drug Discovery Platforms
AI-driven drug discovery platforms are changing the game by speeding up the design and development of new treatments. Companies like Exscientia have showcased the power of AI in drug design. Their Centaur Chemist platform can create molecules faster than traditional methods, as seen when Exscientia developed an AI-designed cancer drug that entered clinical trials within a remarkable year.
The impact of these AI platforms is profound. They can reduce drug discovery costs by up to 40% and slash development timelines from five years to as little as 12-18 months. By streamlining the screening process and quickly identifying promising candidates, AI is helping biopharma companies bring new therapies to market faster and more efficiently.
New Advancements of Generative AI in Molecular Design
Generative AI is making huge strides in molecular design, especially with models like AlphaFold and the newer Genie. These advancements are reshaping how we approach protein folding and drug development, opening doors to faster, more accurate solutions.
AlphaFold predicts protein structures with remarkable accuracy from amino acid sequences. This breakthrough has accelerated progress in drug development and biology, helping researchers tackle challenges like malaria, cancer, and even creating plastic-digesting enzymes. Over 1.2 million researchers worldwide are already using AlphaFold to drive these innovations.
But it doesn’t stop there. Newer models like Genie are taking things a step further. Instead of just predicting protein structures, Genie can now design entirely new proteins that don’t exist in nature. This ability to create custom proteins opens up exciting possibilities for faster drug discovery and treatments tailored to specific needs.
These generative AI models are also making it easier to design drugs by predicting how potential treatments will interact with protein targets. This is helping researchers refine drug development processes, particularly for complex diseases like Alzheimer’s and cancer.
AI Impacts Clinical Trial Decentralization
Decentralized Clinical Trials (DCTs) are quickly becoming a game-changer in the world of clinical research. Supported by AI, these trials are breaking down barriers to participation, making clinical studies more accessible to diverse populations across the globe. No longer do patients have to travel long distances or face logistical hurdles to participate in trials. The FDA has recognized the benefits of DCTs, citing improvements in convenience, patient recruitment, retention, and overall productivity. In fact, DCTs are now at the forefront of advancing clinical trials, particularly in underserved communities.
But the real magic happens when AI steps in to streamline and enhance the process. One of the most significant areas where AI is making a difference is patient recruitment. AI algorithms, combined with vast datasets from electronic health records (EHRs) and real-world data (RWD), are transforming how participants are identified and matched with clinical trials. By analyzing detailed patient data—such as medical history, symptoms, and demographic information—predictive models can pinpoint eligible participants with remarkable speed and accuracy. What used to take weeks or even months can now be accomplished in just days.
This is a major win for clinical trials, where up to 25% of studies fail due to insufficient enrollment. With AI reducing recruitment times, the chances of completing trials on time and with adequate participation have greatly improved, ultimately accelerating the development of new treatments. In this way, AI isn’t just optimizing the process—it’s making clinical trials more inclusive, diverse, and accessible to a broader range of participants.
Use of Intelligent Automation in Drugs Manufacturing
The future of drug manufacturing is quickly becoming intertwined with intelligent automation, and the numbers say it all. According to a PwC survey, 79% of pharmaceutical executives believe that intelligent automation will have a significant impact on their industry in the next five years. But what does this mean in practice? In simple terms, it’s transforming the way drugs are made, moving from a traditional, manual approach to a highly efficient, AI-driven system.
Take a look at Pfizer’s use of intelligent automation to streamline its temperature-controlled logistics. Drug production is a delicate process, especially when it comes to maintaining the right conditions for sensitive products. With automation, Pfizer has been able to reduce errors, boost efficiency, and optimize the entire supply chain—from raw materials to finished products. This isn’t just about saving time; it’s about improving the quality of the drugs, lowering operational costs, and ensuring that patients get the best possible treatment outcomes.
But the benefits don’t stop at logistics. AI-powered automation is also stepping in to predict when equipment needs maintenance. It’s a bit like having a crystal ball for your machinery—by predicting potential failures before they happen, manufacturers can avoid costly downtime and keep production flowing smoothly. This predictive approach not only saves money but also ensures that production schedules stay on track, avoiding delays that could impact drug availability.
And here’s where things get even more exciting: digital twins. These virtual models of manufacturing processes are starting to take center stage in drug production. By simulating the entire manufacturing process in real time, digital twins allow companies to test scenarios and identify potential inefficiencies before they happen. Unlearn, for example, used digital twins in a study on Alzheimer’s disease, reducing the number of participants required by 35% in control arms and 21% for the overall study. This kind of efficiency could shave years off drug development timelines, making it faster to bring life-saving treatments to market.
Challenges and Opportunities of AI in Pharma & Biotech Industry
As AI continues to shape the pharmaceutical and biotechnology sectors, it brings both significant challenges and promising opportunities. Below, we explore key aspects of the industry where these challenges and opportunities intersect.
Aspect | Proof of Concept (PoC) | Prototype |
Drug Discovery | • High data quality requirements • Complex biological data integration • Lack of interpretability in AI decisions (black-box nature) |
• Faster identification of potential drug candidates • More efficient screening of compound libraries • Accelerated lead optimization |
Clinical Trials | • Regulatory hurdles • Patient privacy concerns • Bias in data affecting trial outcomes |
• Streamlined patient recruitment through predictive algorithms • Decentralized and remote trials for broader participation |
Data Availability |
• Incomplete or noisy biological datasets |
• AI can leverage existing public datasets and synthesize novel data for new insights |
Regulatory Compliance | • Lack of standardized guidelines for AI-based drug approval • AI model transparency |
• Potential for new regulatory frameworks tailored to AI-driven drug development |
Talent and Expertise | • Shortage of professionals with both AI and biological expertise | • Growing demand for interdisciplinary roles blending AI, bioinformatics, and pharmaceutical sciences |
Manufacturing | • Complex scaling processes • Integration of AI systems with existing infrastructure |
• AI-driven automation improves precision and reduces operational costs in drug production and logistics |
Partnerships | • Difficulty aligning tech culture with traditional pharma workflows | • Increasing collaborations between AI firms and biopharma can accelerate innovation |
Personalized Medicine | • Need for real-time patient data and high computation power | • AI enables personalized treatment plans based on genetic, phenotypic, and environmental data |
Predictive Maintenance | • Integrating AI tools with older equipment • High upfront costs |
• Reduces downtime by anticipating equipment failures • Optimizes drug supply chain management |
Regulatory and Ethical Considerations of AI in Biotech
As AI makes its mark in biotech, it brings both exciting possibilities and complex challenges for regulatory and ethical frameworks. In this section, we explore how regulatory bodies are navigating these hurdles and what ethical practices are needed for AI to truly benefit patients.
AI and Regulatory Bodies
As AI increasingly impacts drug development, regulatory agencies like the FDA and EMA are stepping up to ensure safety and efficacy. The FDA, recognizing AI’s transformative potential, has introduced initiatives like the Software Pre-Certification Program to streamline the approval process for AI-driven drugs and devices. But AI presents unique challenges. Many models operate as “black boxes,” making it difficult for regulators to fully understand or explain their decision-making. This raises concerns about transparency and accountability in drug approvals.
Balancing rapid innovation with rigorous safety protocols is no easy feat. Regulatory bodies must craft frameworks that allow AI to advance without compromising public health. This requires flexibility as AI technologies evolve. While these agencies work on refining guidelines, their role remains pivotal in ensuring that AI contributes to breakthroughs without creating new risks for patients. The future will likely see more streamlined pathways for AI-based solutions, but patient safety must remain a priority.
AI in Ethical Drug Development
The WHO has highlighted AI’s potential to accelerate pharmaceutical progress, yet bias in AI algorithms poses a significant risk. Unequal healthcare outcomes could result if AI models are not representative of all populations, leading to treatments that work for some but not for others.
Ensuring ethical AI use means demanding transparency in decision-making. Regulatory bodies are increasingly focused on this, pushing for AI systems that are clear, explainable, and fair. AI can also improve drug safety by enhancing supply chain traceability, as seen with the U.S. Drug Supply Chain Security Act (DSCSA). Ultimately, AI must be used responsibly to ensure it benefits all patients equitably.
Successful Case Studies of AI in Pharma Industry
AI is already proving its transformative impact in the pharma industry. Successful case studies like AlphaFold and Novartis highlight how AI is transforming research and production, bringing tangible benefits to the field.
AI in Drug Discovery
AI is rapidly reshaping drug discovery, and AlphaFold is a perfect example of its potential. This AI-powered tool predicts protein structures with almost the same accuracy as traditional experimental methods. In fact, during the prestigious CASP14 competition, AlphaFold achieved a jaw-dropping median backbone accuracy of 0.96 Å—so close to the real structure it’s almost uncanny. This breakthrough allows researchers to understand complex proteins faster, which is crucial for designing effective drugs. With its ability to handle long proteins and accurately predict their structure, AlphaFold is giving drug developers an edge, speeding up the discovery of treatments for diseases like Alzheimer’s and cancer.
AI in Biopharma Manufacturing
Novartis is leading the charge with AI-driven analytics that monitor production in real time. These smart systems can detect quality issues before they become costly problems, reducing waste and minimizing human error. By automating quality checks, Novartis is able to maintain higher standards and ensure that every batch of medicine meets strict safety requirements. The result? Faster, more reliable production that ensures patients get the best possible treatments, every time.
Accelerate Your Pharma R&D with AI Technology
Get in touch with Coherent Solution to begin your AI-driven transformation in Pharma R&D.
AI in Biopharma Beyond 2024
Looking ahead, AI’s role in biopharma will expand exponentially, especially when combined with emerging technologies like synthetic biology and quantum computing. AI’s ability to process massive datasets will be further amplified by quantum computing, making it possible to tackle more complex biological systems. Together, these technologies hold the key to solving long-standing challenges like drug resistance and the inefficiencies of traditional research and development methods.
As the healthcare industry shifts towards patient-centric models, AI will play a central role in improving personalized care. AI-powered wearables and predictive healthcare tools will enable continuous monitoring of patients, allowing for early disease detection and proactive intervention. This move toward ongoing care could revolutionize the way we manage chronic conditions and overall health.
In drug development, AI will continue to drive faster, more efficient discovery processes. By analyzing data, AI is already uncovering new therapeutic targets, optimizing clinical trial designs, and predicting patient responses with greater accuracy. This trend will only intensify, leading to quicker, more successful drug development cycles. With AI accelerating the pace of innovation, the future of biopharma looks brighter, delivering more personalized treatments, faster cures, and improved patient outcomes.
Coherent Solutions: A Reliable AI Development Partner for Biopharma
At Coherent Solutions, we offer custom AI solutions designed to optimize every aspect of biopharma operations. With over 30 years of experience and a team of 100+ AI and data analytics experts, we have the expertise to transform complex challenges into breakthrough solutions. Our work with Fortune 500 companies highlights our proven ability to deliver cutting-edge biotech software that drives results.
Ready to take your biopharma operations to the next level? Reach out today to get started.
Frequently Asked Questions
-
AI accelerates drug discovery by predicting molecule interactions, reducing timelines and costs by up to 50% in preclinical stages.
-
The AI in pharmaceutical market is expected to grow from $1.94B in 2025 to $16.49B by 2034, with a CAGR of 27%.
-
Yes, Pfizer, Moderna, and Johnson & Johnson are leveraging AI for drug development, clinical trials, and operational efficiencies.
-
AI streamlines patient recruitment, improves trial design, and enhances data analysis, leading to faster, more reliable results.
-
Key concerns include data privacy, algorithm transparency, and bias mitigation—leading to increased regulatory focus on AI governance.