According to a survey by Komprise, around 68% of enterprises allocate nearly 30% of their IT budget to data storage, management, and protection. This proves that they recognize the importance of data governance in their digital transformation journey. Effective data management is now a necessity. It allows organizations to make informed decisions, streamline operations, and remain competitive.
This article explores best practices and frameworks for AI-enabled data governance. Discover how AI can elevate data quality, security, and compliance, ultimately simplifying data management and maximizing its potential.
What is Data Governance?
Data governance is a structured approach to managing information throughout its lifecycle. It defines processes, roles, and policies to ensure data is used efficiently and responsibly. In essence, it ensures that the right people can take the right actions with data under the right conditions.
It is often confused with data management. While data management focuses on the technical aspects of handling data, such as storage and processing, data governance focuses on the strategic aspects like oversight and control. Data governance answers the “why” and “how” of data use, while data management addresses the “what” and “where.”
Key components of data governance include:
- Data Quality: Ensuring it is accurate, complete, and trustworthy.
- Data Stewardship: Assigning clear roles and responsibilities for data ownership and oversight.
- Data Security: Protecting data from unauthorized access and breaches.
- Compliance: Adhering to legal and regulatory data standards.
- Data Lifecycle Management: Defining how data is created, maintained, archived, and deleted.
- Policies and Standards: Establishing clear rules for data handling, including collection, storage, access, use, and sharing.
The Importance of Data Governance
Imagine trying to navigate a city without any traffic rules. Chaos, right? That’s what managing data without governance looks like. With the rise of AI, data governance has become even more essential. It’s no longer just a nice-to-have; it’s a must.
No matter how big your business is, you can’t afford to ignore it. With growing regulations and the constant threat of cyberattacks, the stakes are higher than ever. But beyond compliance, well-implemented data governance can be the secret sauce that enhances decision-making and boosts operational efficiency. Let’s explore why it’s a game changer:
- Regulatory Compliance: It helps organizations meet strict regulations and avoid penalties.
- Improved Decision-Making: Well-managed, high-quality data leads to more accurate insights and better business decisions.
- Risk Mitigation: It reduces risks associated with data breaches and misuse.
- Operational Efficiency: Clear policies and procedures streamline data-related processes.
- Enhanced Data Quality: Governance practices ensure it is accurate, consistent, and reliable.
- Trust and Transparency: It builds trust with customers and stakeholders by demonstrating responsible data handling.
Data governance isn’t just a box to check—it’s an investment in your company’s future. Remember, in today’s digital age, the real power lies in how well you govern your data. With best practices and frameworks, you not only protect your organization from risks but also position it to thrive in a data-driven world.
Challenges in Traditional Data Governance
While data governance using AI offers numerous benefits, some companies still rely on traditional methods. However, affected by strict policies and standards, they face challenges that limit their effectiveness.
One major challenge is the complexity and bureaucracy of traditional systems, which often result in slow, cumbersome processes that hinder the ability to adapt quickly.
This can be further complicated by data silos, when information is isolated across departments, which causes inconsistencies and inefficiencies across the organization.
Moreover, traditional systems lack the flexibility to keep up with new technologies and changing business needs. As regulatory requirements change, these methods may not remain relevant either.
Furthermore, the sheer volume and variety of data generated today can overwhelm traditional governance models, especially when paired with outdated technology that limits their overall effectiveness.
AI Maturity Assessment: A Crucial Step
According to Accenture, only 12% of firms have achieved a level of AI maturity that leads to superior growth and transformation, underscoring the challenges many face in effectively harnessing AI technologies. And while businesses are actively turning to AI-enabled data governance, its effective implementation doesn’t come without challenges.
To navigate them, organizations must assess their AI maturity. Why is this so important?
Simply put, without a thorough assessment of an organization’s readiness for AI, even the most advanced technologies can lead to ineffective implementation, reduced data quality, increased risk, and wasted resources. Think of it like building a skyscraper without first ensuring the foundation is solid.
An AI maturity assessment allows companies to evaluate their readiness to implement AI across multiple dimensions, providing a solid foundation before full-scale AI integration. This approach not only helps reduce risks but also allows organizations to maximize the return on their AI investments.
So, what should businesses focus on when conducting an AI maturity assessment? Here are the key components:
- Data Management Capabilities: Evaluating how well an organization manages its data.
- Technology Infrastructure: Assessing the technological foundation needed to support AI.
- Skill and Talent Readiness: Measuring the availability of skilled personnel.
- Process and Policy Alignment: Ensuring alignment between organizational processes and AI initiatives.
- Ethical and Regulatory Compliance: Reviewing adherence to ethical standards and regulations.
- Cultural Readiness: Gauging the organization’s openness to adopting AI.
Request AI Maturity Assessment for Your Business
Let Coherent Solutions' experts assess your AI maturity for successful data governance implementation.
Request a free consultationComparing AI-Powered and Traditional Approaches
AI technologies have become a revolution in recent years. They are implemented in various sectors, and data governance is no exception. Compared to traditional governance which relies on manual processes, AI-driven models offer automation and advanced analytics. With growing data volumes and strict regulations, organizations have to choose between these approaches.
Let’s compare the key differences between traditional methods with the innovative solutions AI brings to the table.
Aspect | AI-Powered Data Governance | Traditional Data Governance |
---|---|---|
Complexity Management | Automates complex tasks such as data classification and policy enforcement. | Often involves manual processes, leading to higher complexity and potential errors. |
Data Integration | Automatically integrates and harmonizes data from diverse sources. | Typically requires manual integration, leading to data silos and inconsistencies. |
Adaptability | Adapts to new data sources and evolving regulations dynamically. | Less flexible; changes often require significant manual adjustments. |
Data Quality | Continuously monitors and improves data quality using machine learning. | Relies on periodic manual checks and remediation for data quality issues. |
Compliance Monitoring | Real-time monitoring and automated compliance checks with alerts. | Compliance checks are often periodic and may be slower to detect issues. |
Data Privacy | Automated identification and protection of sensitive data, applying encryption and access controls. | Privacy measures are typically manual and less responsive to changing data needs. |
Data Lineage | AI tools provide clear, automated tracking of data lineage and transformations. | Tracking is often manual, which can be error-prone and less comprehensive. |
Scalability | Scales efficiently with large volumes and varieties of data. | Scaling up can be challenging and resource-intensive. |
User Training and Onboarding | AI tools often require specialized training but provide more user-friendly interfaces. | Traditional systems might be less intuitive, requiring extensive training. |
Risk Management | Proactively predicts and manages risks through predictive analytics. | Risk management is reactive, often dealing with issues after they arise. |
Transparency | Provides explainable AI models and detailed documentation of decision-making. | Often lacks transparency in how decisions are made and data is managed. |
Operational Efficiency | Automates routine tasks, reducing manual effort and increasing efficiency. | Many tasks are manual, leading to higher labor costs and potential inefficiencies. |
The Integration of AI into Data Governance Practices
Integrating AI into data governance changes how organizations manage and protect their information. In this regard, we can compare AI governance vs data governance as two distinct yet related concepts. Understanding how these disciplines are tied will help organizations better structure their practices. It will guarantee the ethics, efficacy, and reliability of AI implementation.
AI governance refers to managing the AI systems within the organization. It guarantees they are used responsibly and ethically. This includes monitoring the behavior of AI models and ensuring they align with legal and ethical standards. On the other hand, AI data governance focuses specifically on managing data used by AI systems. It’s crucial because the behavior and performance of AI models are directly impacted by the data used to train them and which they operate. The discipline ensures data accuracy and security, as well as following the regulations.
Both are integral components of IT governance and corporate governance. They ensure that organizations not only comply with legal and regulatory requirements but also maintain ethical standards in their AI initiatives. By integrating these governance frameworks, organizations can create a culture of accountability and transparency, ultimately increasing credibility with partners.
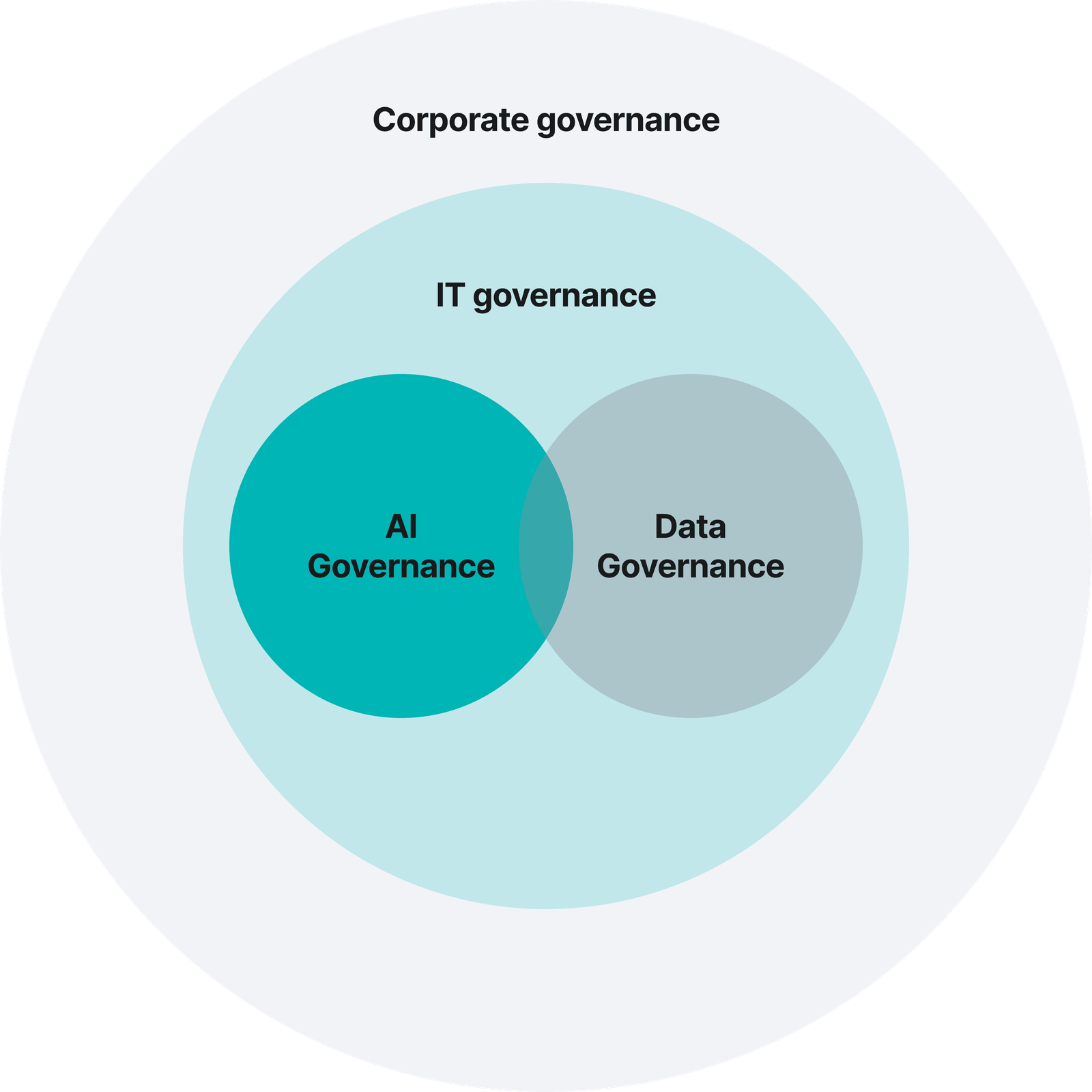
AI Technologies Powering Data Governance
Organizations leverage a variety of AI technologies to streamline and enhance their data governance practices:
- Machine Learning (ML): The machine learning data governance algorithms automate data quality checks, detect anomalies, and predict trends. They ensure its accuracy and reliability by identifying patterns and inconsistencies in large datasets.
- Natural Language Processing (NLP): NLP tools analyze and interpret unstructured data, such as emails and documents. They simplify data classification, labeling, and information extraction, improving its accessibility and management.
- Predictive Analytics: Predictive analytics uses statistical algorithms and ML techniques to forecast future trends and potential data risks. This enables organizations to make proactive decisions and manage risk effectively.
- Automated Data Discovery and Cataloging: AI-powered tools can automatically scan and catalog data assets, creating comprehensive registers. This facilitates data management and control by providing information on data origin, use, and compliance.
- Robotic Process Automation (RPA): RPA automates repetitive data governance tasks such as data entry and report generation. This reduces human error, increases efficiency, and ensures consistent practices.
- AI-driven Data Privacy Management: AI technologies monitor and enforce data privacy policies, automatically identifying sensitive data and ensuring compliance with regulations like GDPR or CCPA. This reduces the risk of data breaches and non-compliance.
- Intelligent Data Lineage: AI can track the origin, transformations, and movement of data throughout its lifecycle, maintaining accountability and transparency in data governance processes.
Data Governance and Machine Learning
Machine learning is a cornerstone of most AI technologies. Its algorithms automate and improve many governance processes.
Firstly, it can efficiently handle massive volumes of data that would be impossible to manage manually. Secondly, its algorithms consistently outperform humans in accuracy and precision. This automation of routine tasks frees up valuable human resources for more strategic work. Moreover, ML models can learn and adapt to evolving data environments and changing governance requirements. Lastly, while the initial investment in data governance using machine learning may be substantial, it can lead to significant long-term cost savings.
Benefits of Incorporating AI into Data Governance
Incorporating AI in data governance opens up a world of exciting possibilities for organizations. With the help of AI software solutions, they can streamline processes and improve their ability to manage and analyze data effectively. Let’s explore how this cutting-edge technology can revolutionize the way you govern data:
- Reduced Bureaucratic Burden: AI automates routine governance tasks, streamlining processes and reducing administrative overhead.
- Automated Data Integration: AI-powered tools can automatically discover, catalog, and integrate data from various sources.
- Adaptability: Machine learning models adapt to changing data patterns and governance requirements over time.
- Regulatory Changes Monitoring: AI can monitor and interpret regulatory changes, helping organizations stay compliant.
- Big Data Processing: AI technologies enable effective governance of large-scale, complex datasets.
- Advanced Analytics: AI brings sophisticated analytical capabilities to data governance, enabling deeper insights and more nuanced decision-making.
Use Cases and Applications of AI in Data Governance
AI technologies help organizations manage their data assets more efficiently and effectively. Beyond theory, let’s explore how AI transforms data governance in practice. We’ll consider the possible use cases, as well as practical applications.
Use case | Application |
---|---|
Automated Data Classification and Tagging | AI-driven tools automatically categorize and tag data based on content and context. |
Data Quality Management | Machine learning algorithms identify and correct data inconsistencies, duplicates, and errors. |
Predictive Data Governance | Advanced models forecast potential data governance issues, enabling proactive management. |
Enhanced Data Discovery and Cataloging | AI tools automatically discover, index, and catalog data assets for a unified view. |
Data Privacy Management | Artificial intelligence identifies sensitive data, applies encryption, and enforces access controls automatically. |
Intelligent Metadata Management | AI generates and updates metadata, improving data discoverability and usability. |
Automated Data Lineage Tracking | AI visualizes data lineage, showing data flow and transformations across systems. |
Dynamic Policy Enforcement | Data governance policies are adjusted dynamically based on data patterns and regulatory changes. |
Fraud Detection and Prevention | AI detects unusual data access patterns or transactions, flagging potential fraud. |
AI for Data Governance: Implementation Strategy
Integrating AI into data governance may seem complex, but breaking the process into clear phases makes it manageable. Here’s a structured, step-by-step guide to help you navigate.
Phase 1: Laying the Groundwork
The foundation of successful AI-powered data governance begins with clear planning.
First, identify your needs and set goals. Begin by pinpointing your organization’s challenges and setting measurable data governance goals supporting your business objectives.
Next, evaluate the existing system and identify gaps. Assess your current systems to identify strengths, weaknesses, and areas for improvement. This understanding will help select the right AI technology.
Phase 2: Selecting and Implementing the Tools
With a clear understanding of your needs and system gaps, it’s time to find the right AI tools and establish a governance framework.
Start with choosing the right AI technologies. Research and select AI solutions that address your specific needs. Consider factors like scalability, integration capabilities, and vendor support.
Then, develop a comprehensive AI data governance framework. Define roles, responsibilities, policies, and procedures, ensuring alignment with compliance requirements and strategic objectives.
Phase 3: Integration and Training
Now, it’s time to put the plan into action by integrating the selected AI tools and aligning them with your operations.
Integrate your chosen technologies into your existing framework, customizing them to fit your specific processes and needs.
Also, train and onboard your staff. According to a Deloitte survey, 68% of executives report a moderate-to-extreme skills gap in AI implementation. Provide adequate training and support to your staff, ensuring they understand how to utilize the new AI tools effectively.
Phase 4: Monitoring, Compliance, and Scaling
Once AI tools are in place, continuous improvement and adherence to regulations are key to long-term success.
Establish metrics to track performance and gather regular feedback from users. It will help find the areas for improvement.
Furthermore, prioritize compliance with data protection regulations and security standards. Regularly review your practices to ensure they meet legal requirements.
Finally, scale up and innovate. Once established, look for opportunities to scale up your AI efforts. Explore innovative technologies and methodologies, gradually expanding AI implementation.
Empower your organization with AI-enabled data governance. Start small, think big, and act fast to transform your practices. The future of efficient, accurate, and compliant data governance awaits your action.
Best Practices for AI Implementation
Successfully implementing AI technologies involves following key practices that ensure effective integration, maximize benefits, and minimize potential risks. Here are some best practices to consider:
- Pilot Projects and Gradual Expansion: Start with small-scale pilots to test AI applications in a controlled environment before full-scale rollout. Gradually expand the scope based on the pilot’s success to minimize risks and ensure smooth integration.
- Data Preparation and Quality Maintenance: Invest in thorough data preparation to ensure its quality, avoiding biases and inaccuracies in data governance artificial intelligence models. Regularly update and maintain data quality to avoid biases and inaccuracies.
- Cross-Functional Teams and Stakeholder Engagement: Form teams with diverse expertise to ensure robust AI solutions. Engage stakeholders early and often to align AI initiatives with business goals.
- Explainable AI and Thorough Documentation: Use transparent AI models and thoroughly document AI models, data sources, and decision-making processes.
- Data Protection and Access Controls: Implement robust protection measures and access controls to safeguard sensitive information. It will help restrict access to authorized personnel only, maintain privacy, and comply with regulations.
- Regularly Review and Update AI Models: Regularly review and update AI models to maintain effectiveness and adapt to changing conditions.
Implement AI Data Governance Seamlessly with Coherent Solutions
Reach out to Coherent Solutions and get top-tier AI data governance implementation services.
Request a free consultationAI-Powered Data Governance Frameworks
Does your organization strive to govern data with AI technologies? Consider frameworks that can offer guidance and provide best practices. Here’s a brief overview of the six influential ones.
The AI Governance Framework by NIST (National Institute of Standards and Technology)
The NIST AI Governance Framework is a vital guide for building innovative and reliable AI systems. It focuses on creating trustworthy, transparent, and accountable AI applications. The emphasis on risk management helps organizations identify potential threats and ensure that AI systems operate fairly and securely.
Trustworthiness is central to the framework. It ensures that AI systems are fair and reliable under various conditions. Risk management is another key element, that identifies and mitigates AI-related risks. Transparency is promoted through clear documentation, making AI operations and decisions easier to explain and understand. Finally, the framework accounts for oversight over AI systems and responsibility for how they are used.
The European Commission’s Ethical Guidelines for Trustworthy AI
These guidelines ensure that AI systems are developed and deployed in line with societal values and human rights. They are built around respect for human autonomy, which promotes the idea that AI should support, not replace, human decision-making.
Furthermore, the guidelines prioritize the prevention of harm, highlighting the need for AI systems to mitigate risks that could impact individuals or society. Fairness is another crucial aspect, requiring AI systems to be non-discriminatory and fair. Transparency is another key pillar, with the guidelines demanding clear and understandable AI operations.
The FAIR Principles (Findable, Accessible, Interoperable, Reusable)
The FAIR principles are the backbone of effective data management in AI governance. They ensure that data is not just stored but structured in a way that makes it discoverable and usable across various systems.
One of their main goals is to make data findable by using proper identifiers and metadata. Accessibility is also a key priority, as data must be available under clear and understandable protocols and conditions. Moreover, these principles emphasize the importance of interoperability, promoting data that can seamlessly integrate across different platforms and systems. Lastly, the principles stress the importance of well-documented and formatted data to facilitate its reuse.
The Data Management Body of Knowledge (DMBOK)
DMBOK provides a comprehensive approach to managing data assets, offering insights into data governance, quality, architecture, and daily operations. It stresses the importance of strong data governance policies, which define roles, responsibilities, and processes for managing data effectively.
Additionally, it highlights the need for data quality management, ensuring that data is accurate, reliable, and consistent. Data architecture is another critical component, as it outlines the organization and structure of data systems within the organization. Moreover, it also addresses the practical aspects of data governance, offering insights into managing daily AI and data governance activities.
The AI Ethics Guidelines by the IEEE (Institute of Electrical and Electronics Engineers)
The IEEE’s guidelines provide a roadmap for integrating ethical considerations into AI system design. They go beyond mere compliance, encouraging organizations to embed ethical considerations into every stage of AI development. The guidelines also stress the importance of safeguarding user data and privacy, ensuring that AI systems respect individual rights. In addition, they advocate for clear accountability in AI outcomes, promoting transparency in AI decision-making processes.
The CDO (Chief Data Officer) Council’s Data Governance Framework
The CDO Council framework highlights the strategic role of the Chief Data Officer in overseeing data governance. The framework presents an instruction for developing data strategies that are fully aligned with the organization’s goals. It focuses on defining data ownership and offers guidance on determining responsibilities for data stewardship, ensuring that data ownership is clearly defined within the organization.
The framework also helps in developing comprehensive data security, compliance, and governance policies. Data strategy is another key area, as it involves aligning data governance initiatives with broader business goals to improve the overall organizational performance. Perhaps most importantly, it emphasizes the need to use performance metrics to measure and continuously improve governance effectiveness.
Key Policies of AI-Powered Data Governance Frameworks
These frameworks rely on key policies to ensure the responsible and effective use of AI technologies. These policies define data privacy, accountability, compliance, and ethical use of AI. Let’s take a look at some of the key ones that support transparency, risk management, and compliance.
Policy | Overview | Key Elements |
---|---|---|
Data Classification and Management | Establishes how data should be categorized and managed based on sensitivity and usage. | Automated Classification: AI categorizes data into classes. Data Tagging: AI tags data with metadata. Policy Review: Regularly update classification criteria. |
Data Quality Management | Ensures data accuracy, completeness, consistency, and timeliness using automated processes. | AI-Driven Data Quality: AI monitors data quality in real time. |
Data Privacy and Security | Protects sensitive data from unauthorized access and breaches with AI-enhanced measures. | AI-Based Privacy Tools: AI detects and masks sensitive data. |
Compliance and Regulatory Adherence | Ensures adherence to laws and regulations, integrating AI for monitoring and reporting. | Automated Compliance Tracking: AI monitors regulatory changes. |
Transparency and Explainability | Promotes transparency in AI systems and ensures that AI decisions are explainable. | Explainable AI Models: AI provides clear explanations for decisions. |
Ethical Use of AI | Guides ethical AI system development and use, focusing on fairness and accountability. | Bias Detection: AI identifies and mitigates biases. |
Data Lineage and Provenance | Tracks and documents data flow and transformation for integrity and traceability. | Automated Data Lineage Tracking: AI visualizes and tracks data flow. |
Model Management | Governs AI model development, validation, and monitoring to ensure effectiveness and compliance. | AI Model Validation: AI validates model accuracy. |
Risk Management | Identifies, assesses, and mitigates risks related to data and AI systems using predictive tools. | Predictive Risk Assessment: AI forecasts and assesses risks. |
Training and Awareness | Provides training and resources to ensure stakeholders are knowledgeable about data governance and AI. | AI-Driven Training Programs: Personalized training with AI. |
Incident Response and Remediation | Defines procedures for addressing data governance incidents with AI-enhanced response mechanisms. | AI-Powered Incident Detection: Monitor for anomalies. |
Advance Your Data Governance Strategy with Coherent Solutions
Coherent Solutions offers effective solutions to help you unlock the full potential of your data.
Take the next step towards smarter, safer data management. Contact us today to learn how our AI-powered data governance solutions can transform your organization. Our experts will work closely with you to implement industry-leading practices that optimize data value, reduce risk, and drive sustainable growth.